Graph Neural Networks
Contents
8. Graph Neural Networks¶
æŽå²çã«ãæ©æ¢°åŠç¿ã§ååãæ±ãéã®æå€§ã®èª²é¡ã¯ãèšè¿°åã®éžå®ãšãã®èšç®ã§ãããã°ã©ããã¥ãŒã©ã«ãããã¯ãŒã¯ïŒGNNïŒã¯ãã°ã©ããå ¥åãšãããã£ãŒããã¥ãŒã©ã«ãããã¯ãŒã¯ã®äžçš®ã§ãããGNNã¯ååãçŽæ¥å ¥åãšããŠåãåãããšãã§ãããããèšè¿°åã«é ãæ©ãŸããå¿ èŠããããŸããã
æ¬ç« ã®æ³å®èªè ãšç®ç
ãã®ç« ã§ã¯ãStandard Layers ãš Regression & Model Assessment ã®å 容ã¯åæãšããŠããŸããæ¬ç« ã§ã¯ã°ã©ããGNNã®å®çŸ©ãã説æããŸãããã°ã©ãããã¥ãŒã©ã«ãããã¯ãŒã¯ã®åºæ¬çãªæŠå¿µã«ã€ããŠäºãæ £ããŠãããšãªãè¯ãã§ãããããã®ç« ãåŠã¶ããšã§ã以äžãã§ããããã«ãªããŸãïŒ
ååãã°ã©ãã§è¡šçŸã§ãã
äžè¬çãªGNNã®ã¢ãŒããã¯ãã£ãè°è«ããããGNNã®çš®é¡ãçè§£ã§ãã
GNNãæ§ç¯ããã©ãã«ã®çš®é¡ã«å¿ããèªã¿åºã颿°ïŒread-out functionïŒãéžæã§ãã
ã°ã©ãããšããžãããŒãã®ç¹åŸŽãããããåºå¥ã§ãã
GNNããšããžæŽæ°ãããŒãæŽæ°ãéçŽã®åã¹ãããã«åããŠå®åŒåã§ãã
GNNã¯ã°ã©ããå ¥åããã³åºåããããã«ç¹å¥ã«èšèšãããã¬ã€ã€ãŒã§ããGNNã«ã€ããŠã®ã¬ãã¥ãŒã¯è€æ°å·çãããŠãããäŸãã° Dwivedi et al.[DJL+20], Bronstein et al.[BBL+17], Wu et al.[WPC+20] ãªã©ãæããããŸãã GNNã¯ãç²èŠååååååŠã·ãã¥ã¬ãŒã·ã§ã³ [LWC+20] ããNMRã®ååŠã·ããäºæž¬ [YCW20] ãåºäœã®ãã€ããã¯ã¹ã®ã¢ããªã³ã° [XFLW+19] ãŸã§ãããããã¢ããªã±ãŒã·ã§ã³ã«é©çšã§ããŸãã GNNã«ã€ããŠæ·±ãèžã¿èŸŒãåã«ããŸãã°ã©ããã³ã³ãã¥ãŒã¿äžã§ã©ã®ããã«è¡šçŸãããååãã©ã®ããã«ã°ã©ãã«å€æããããçè§£ããŸãããã
ã°ã©ããšGNNã«ã€ããŠã®ã€ã³ã¿ã©ã¯ãã£ããªå ¥éè³æãã distill.pub [SLRPW21] ã§æäŸãããŠããŸããçŸåšã®GNNã®ç ç©¶ã®ã»ãšãã©ã¯ãã°ã©ãã«ç¹åãããã£ãŒãã©ãŒãã³ã°ã©ã€ãã©ãªãçšããŠè¡ãããŠããã2022幎çŸåšæã代衚çãªã©ã€ãã©ãªã¯ PyTorch Geometric, Deep Graph library, Spektral, TensorFlow GNNS ãªã©ã§ãã
8.1. ã°ã©ãã®è¡šçŸÂ¶
ã°ã©ã \(\mathbf{G}\) ã¯ãããŒã \(\mathbf{V}\) ããã³ãšããž \(\mathbf{E}\)ãã®éåã§ãã æã ã®ã»ããã£ã³ã°ã§ã¯ãããŒã \(i\) ã¯ãã¯ãã« \(\vec{v}_i\) ã§å®çŸ©ãããã®ã§ãããŒãã®éåã¯ã©ã³ã¯2ã®ãã³ãœã«ãšããŠè¡šçŸã§ããŸãã ãšããžã¯é£æ¥è¡åïŒadjacency matrixïŒ \(\mathbf{E}\) ã§è¡šçŸããããã \(e_{ij} = 1\) ã§ããã°ããŒã \(i\) ãš \(j\) ããšããžã§çµåããŠãããšã¿ãªãããŸãã ã°ã©ããæ±ãå€ãã®åéã«ãããŠãç°¡åã®ãããã°ã©ãã¯ãã°ãã°æåéå·¡åã°ã©ãïŒãšããžã«ã¯åããããããäžåšããŠå ã®ããŒãã«ã¯æ»ããªãïŒã§ãããšä»®å®ãããŸããããããååã«ãããŠçµåã«ã¯åããç¡ãã茪ãæã€ïŒå·¡åããïŒå Žåãããããšã«æ³šæããŠãã ãããååŠçµåã«ãããŠåãã®æŠå¿µã¯ãªãããšãããæã ãæ±ã飿¥è¡åã¯åžžã«å¯Ÿç§°ïŒ\(e_{ij} = e_{ji}\)ïŒãšãªããŸãããŸãããã°ãã°ãšããžèªèº«ãç¹åŸŽãæã€å Žåãããã \(e_{ij}\) èªäœããã¯ãã«ãšããããšã§è¡šçŸããŸãããã®å Žåã¯é£æ¥è¡åã¯ã©ã³ã¯3ã®ãã³ãœã«ãšãªããŸãããšããžç¹åŸŽã®äŸãšããŠã¯ãå ±æçµåã®æ¬¡æ°ãã2ã€ã®ããŒãéã®è·é¢ïŒïŒååéè·é¢ïŒãªã©ãæããããŸãã
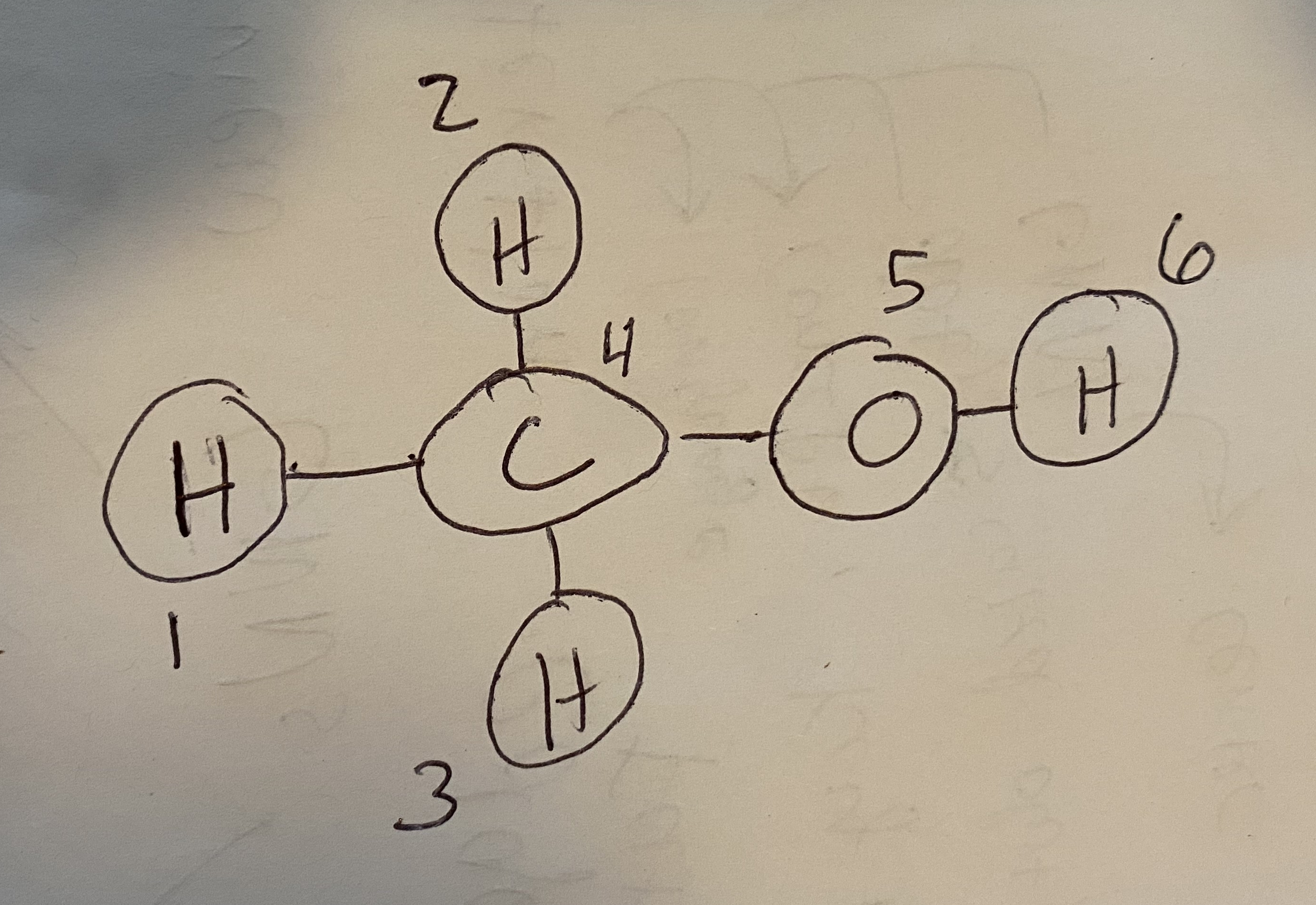
Fig. 8.1 ã°ã©ãã«å€æã§ãããããã¡ã¿ããŒã«ã®åååã«çªå·ãå²ãåœãŠã¶
ã§ã¯ãååããã©ã®ããã«ã°ã©ããæ§ç¯ã§ãããèŠãŠã¿ãŸããããäŸãšããŠãã¡ã¿ããŒã«ãèããŸãïŒ Fig. 8.1 ïŒãããŒããšãšããžãå®çŸ©ããããã䟿å®çã«åååã«çªå·ãæ¯ããŸããããŸãã¯ããã¯ããŒãç¹åŸŽãèããŸããããŒãã®ç¹åŸŽéã«ã¯äœã䜿ã£ãŠãè¯ãã®ã§ãããå€ãã®å Žåãone-hotãšã³ã³ãŒãã£ã³ã°ãããç¹åŸŽãã¯ãã«ã䜿ãããšã«ãªãã§ãããïŒ
Node |
C |
H |
O |
---|---|---|---|
1 |
0 |
1 |
0 |
2 |
0 |
1 |
0 |
3 |
0 |
1 |
0 |
4 |
1 |
0 |
0 |
5 |
0 |
0 |
1 |
6 |
0 |
1 |
0 |
\(\mathbf{V}\) ãããããã®ããŒãã«ã€ããŠã®çµåãããç¹åŸŽãã¯ãã«ã«ãªããŸãããã®ã°ã©ãã®è¿æ¥è¡å \(\mathbf{E}\) ã¯æ¬¡ã®ããã«ãªãã§ãããïŒ
1 |
2 |
3 |
4 |
5 |
6 |
|
---|---|---|---|---|---|---|
1 |
0 |
0 |
0 |
1 |
0 |
0 |
2 |
0 |
0 |
0 |
1 |
0 |
0 |
3 |
0 |
0 |
0 |
1 |
0 |
0 |
4 |
1 |
1 |
1 |
0 |
1 |
0 |
5 |
0 |
0 |
0 |
1 |
0 |
1 |
6 |
0 |
0 |
0 |
0 |
1 |
0 |
å€å°æéããããŠãããã®ã§ãããã2ã€ããã£ãããšçè§£ããŠãã ãããäŸãã°ã1,2,3è¡ç®ã«ã€ããŠã¯ã4åç®ã®æåã ãã0ã§ãªãããšã«æ³šç®ããŠãã ãããããã¯ãåå1ã3ã¯ççŽ ïŒåå4ïŒã«ã®ã¿çµåããŠããããã§ãããŸããååã¯èªåèªèº«ãšã¯çµåã§ããªãã®ã§ãå¯Ÿè§æåã¯åžžã«0ã«ãªããŸãã
ååã ãã§ãªããçµæ¶æ§é ã«ã€ããŠãäŒŒãæ¹æ³ã§ã°ã©ãåã§ããŸããããã«ã€ããŠã¯ Xie et al.ã«ãã [XG18] ãåç §ããŠãã ããã
ããã§ã¯ãSMILESã«ããååã®æåå衚çŸãã°ã©ãã«å€æãã颿°ãå®çŸ©ãããšããããå§ããŸãããã
8.2. ãã®ããŒãããã¯ã®åããæ¹Â¶
ãã®ããŒãžäžéšã® Â Â ãæŒããšããã®ããŒãããã¯ãGoogle Colab.ã§éãããŸããå¿ èŠãªããã±ãŒãžã®ã€ã³ã¹ããŒã«æ¹æ³ã«ã€ããŠã¯ä»¥äžãåç §ããŠãã ããã
Tip
å¿ èŠãªããã±ãŒãžãã€ã³ã¹ããŒã«ããã«ã¯ãæ°èŠã»ã«ãäœæããŠæ¬¡ã®ã³ãŒããå®è¡ããŠãã ããã
!pip install dmol-book
ããã€ã³ã¹ããŒã«ãããŸããããªãå Žåãããã±ãŒãžã®ããŒãžã§ã³äžäžèŽãåå ã§ããå¯èœæ§ããããŸããåäœç¢ºèªããšããææ°ããŒãžã§ã³ã®äžèЧã¯ããããåç §ã§ããŸã
import matplotlib.pyplot as plt
import matplotlib as mpl
import numpy as np
import tensorflow as tf
import pandas as pd
import rdkit, rdkit.Chem, rdkit.Chem.rdDepictor, rdkit.Chem.Draw
import networkx as nx
import dmol
soldata = pd.read_csv(
"https://github.com/whitead/dmol-book/raw/master/data/curated-solubility-dataset.csv"
)
np.random.seed(0)
my_elements = {6: "C", 8: "O", 1: "H"}
äžã®é衚瀺ã»ã«ã§ã¯ã颿° smiles2graph
ãå®çŸ©ããŠããŸãããã®é¢æ°ã¯å
çŽ C, H, Oã«ã€ããŠone-hotãªããŒãç¹åŸŽãã¯ãã«ãçæããŸãããŸãåæã«ããã®one-hotãã¯ãã«ãç¹åŸŽãã¯ãã«ãšãã飿¥ãã³ãœã«ãçæããŸãã
def smiles2graph(sml):
"""Argument for the RD2NX function should be a valid SMILES sequence
returns: the graph
"""
m = rdkit.Chem.MolFromSmiles(sml)
m = rdkit.Chem.AddHs(m)
order_string = {
rdkit.Chem.rdchem.BondType.SINGLE: 1,
rdkit.Chem.rdchem.BondType.DOUBLE: 2,
rdkit.Chem.rdchem.BondType.TRIPLE: 3,
rdkit.Chem.rdchem.BondType.AROMATIC: 4,
}
N = len(list(m.GetAtoms()))
nodes = np.zeros((N, len(my_elements)))
lookup = list(my_elements.keys())
for i in m.GetAtoms():
nodes[i.GetIdx(), lookup.index(i.GetAtomicNum())] = 1
adj = np.zeros((N, N, 5))
for j in m.GetBonds():
u = min(j.GetBeginAtomIdx(), j.GetEndAtomIdx())
v = max(j.GetBeginAtomIdx(), j.GetEndAtomIdx())
order = j.GetBondType()
if order in order_string:
order = order_string[order]
else:
raise Warning("Ignoring bond order" + order)
adj[u, v, order] = 1
adj[v, u, order] = 1
return nodes, adj
nodes, adj = smiles2graph("CO")
nodes
array([[1., 0., 0.],
[0., 1., 0.],
[0., 0., 1.],
[0., 0., 1.],
[0., 0., 1.],
[0., 0., 1.]])
8.3. ã°ã©ããã¥ãŒã©ã«ãããã¯ãŒã¯Â¶
ã°ã©ããã¥ãŒã©ã«ãããã¯ãŒã¯ (GNN) ã¯ã次ã®2ã€ã®ç¹åŸŽãæã€ãã¥ãŒã©ã«ãããã¯ãŒã¯ã§ãã
å ¥åãã°ã©ãã§ãã
åºåã¯é åºç䟡ïŒpermutation equivariantïŒã§ãã
æåã®ç¹ã¯æããã§ããã2ã€ãã®ç¹åŸŽã¯èª¬æãå¿ èŠã§ãããããŸããã°ã©ãã®äžŠã¹æ¿ããšã¯ãããŒããäžŠã¹æ¿ããããšãæå³ããŸãã äžã®ã¡ã¿ããŒã«ã®äŸã§ã¯ãççŽ ãåå4ã§ã¯ãªãåå1ã«ããããšãç°¡åã«ã§ããŸãããã®å Žåãæ°ãã飿¥è¡åã¯æ¬¡ã®ããã«ãªããŸãïŒ
1 |
2 |
3 |
4 |
5 |
6 |
|
---|---|---|---|---|---|---|
1 |
0 |
1 |
1 |
1 |
1 |
0 |
2 |
1 |
0 |
0 |
0 |
0 |
0 |
3 |
1 |
0 |
0 |
0 |
0 |
0 |
4 |
1 |
0 |
0 |
0 |
1 |
0 |
5 |
1 |
0 |
0 |
0 |
0 |
1 |
6 |
0 |
0 |
0 |
0 |
1 |
0 |
ãã®é£æ¥è¡åã®äº€æã«æ£ãã察å¿ããŠGNNã®åºåã倿ãããªãããã®GNNã¯é åºç䟡ã§ãããšèšããŸããéšåé»è·ãååŠã·ããã®ããã«ååããšã«å®çŸ©ãããéãã¢ããªã³ã°ããå Žåããã®ãããªé åºäžå€ã®ä»çµã¿ã¯äžå¯æ¬ ã§ããã€ãŸããããååãå ¥åããé åºãå€ããã°ãäºæž¬ãããéšåé»è·ã®é åºãåæ§ã«å€ãã£ãŠã»ããã®ã§ãã
ãã¡ãããæº¶è§£åºŠããšãã«ã®ãŒã®ããã«ååå šäœã®ç¹æ§ãã¢ããªã³ã°ãããå ŽåããããŸãããããã®éã¯ååã®é çªãå€ããŠã äžå€ïŒinvariantïŒ ã§ããã¹ãã§ããé åºã«ã€ããŠç䟡ïŒequivariantïŒãªã¢ãã«ãäžå€ïŒinvariantïŒã«ãããããåŸã«å®çŸ©ãããªãŒãã¢ãŠãïŒread-outïŒã䜿ããŸããç䟡æ§ã«ã€ããŠã®ãã詳现ãªè°è«ã¯ Input Data & Equivariances ãåç §ããŠãã ããã
8.3.1. ã·ã³ãã«ãªGNN¶
æã ã¯ããããGNNã«èšåããŸãããå®éã«ã¯GNNå šäœã§ã¯ãªãç¹å®ã®ã¬ã€ã€ãŒã®ããšãæããŸããå€ãã®GNNã¯ã°ã©ããåãæ±ãããã«ç¹å¥ã«èšèšãããã¬ã€ã€ãŒãåããŠãããéåžžã¯ãã®ã¬ã€ã€ãŒã«ã€ããŠã®ã¿é¢å¿ãæã¡ãŸããããã§ã¯ãGNNã®ç°¡åãªã¬ã€ã€ãŒã®äŸãèŠãŠã¿ãŸããã:
ãã®åŒã¯ããŸãåããŒãïŒ\(v_{jij}\)ïŒã®ç¹åŸŽã«åŠç¿å¯èœãªéã¿ \(w_{jk}\) ããããåŸãå šãŠã®ããŒãã®ç¹åŸŽãåèšããæŽ»æ§åãé©çšããããšã衚ããŠããŸãããã®æäœã«ãããã°ã©ãã«å¯ŸããŠ1ã€ã®ç¹åŸŽãã¯ãã«ãåŸãããŸããã§ã¯ããã®åŒã¯é åºç䟡ã§ããããïŒçãã¯Yesã§ãããªããªããã®åŒã«ãããŠããŒãã€ã³ããã¯ã¹ã¯ã€ã³ããã¯ã¹ \(i\) ã§ãããåºåã«åœ±é¿ãäžããããšãªãé åºã®äžŠã¹æ¿ããå¯èœã§ããããã§ãã
ã§ã¯æ¬¡ã«ããã®äŸãšäŒŒãŠãããé åºç䟡ã§ã¯ãªãäŸãèŠãŠã¿ãŸãããã
ããã¯å°ããªå€åã§ããããŸãããŒãããšã«1ã€ã®éã¿ãã¯ãã«ããããŸãããããã£ãŠãåŠç¿å¯èœãªéã¿ã¯ããŒãã®é åºã«äŸåããŸããæ¬¡ã«ãããŒãã®é åºãå ¥ãæ¿ãããšãåŠç¿ããéã¿ã¯ããŒãã«å¯Ÿå¿ããªããªããŸãããã£ãŠãããŒãïŒïŒååïŒã®é çªãå€ãã2ã€ã®ã¡ã¿ããŒã«ååãå ¥åãããšãç°ãªãåºåãåŸãããŸããå®éã®ãšããããã®åçŽãªäŸã¯2ã€ã®ç¹ã§å®éã®GNNãšç°ãªããŸãã1ã€ãã¯åäžã®ç¹åŸŽãã¯ãã«ãåºåããŠããŒãããšã®æ å ±ãæšãŠãŠããŸã£ãŠããç¹ã2ã€ãã¯é£æ¥è¡åã䜿çšããªãç¹ã§ããã§ã¯ãé åºç䟡ãç¶æãããã€ããã2ã€ã®æ§è³ªãåããå®éã®GNNãèŠãŠã¿ãŸãããã
8.4. Kipf & Welling GCN¶
åæã«äººæ°ããã£ãGNNã®äžã€ã¯ãKipf & Welling graph convolutional network (GCN) [KW16] ã§ããGCNãGNNã®åºãã¯ã©ã¹ã®äžã€ãšããŠèãã人ãããŸãããæ¬æžã§GCNãšã¯ç¹ã«Kipf & Welling GCNãæããã®ãšããŸãã Thomas Kipfã¯ãGCNã®åªãã玹ä»èšäºãæžããŠããŸãã
GCNã¬ã€ã€ãŒãžã®å ¥åã¯ããŒãããã³ãšããžã®éåïŒè𳿳šïŒåããŒãããã³ãšããžã¯ãã¯ãã«ã§è¡šçŸãããã®ã§ããããã®éåã¯ãã³ãœã«ã§ãïŒ \(\mathbf{V}\), \(\mathbf{E}\) ã§ãåºåã¯æŽæ°ãããããŒãã®éå \(\mathbf{V}'\) ã§ãã åããŒãç¹åŸŽã®æŽæ°ã¯ã \(\mathbf{E}\) ã«ãã衚çŸãããè¿åããŒãã®ç¹åŸŽãã¯ãã«ãå¹³åããããšã§ãªãããŸãã
è¿åããŒãã®æ å ±ãå¹³åããããšã§ãGCNã¬ã€ã€ãŒã¯ããŒãã«ã€ããŠé åºç䟡ã«ãªã£ãŠããŸããè¿åã«ã€ããŠå¹³åãããšããæäœãã®ãã®ã¯åŠç¿ã§ããªãã®ã§ãç¹åŸŽãã¯ãã«ãå ç®ããŠããããŒãã®æ¬¡æ°ïŒçµåããŠããè¿åããŒãæ°ïŒã§é€ç®ããããšã§èšç®ããŸããå¹³åããšãåã«ãåŠç¿å¯èœãªéã¿è¡åãè¿åç¹åŸŽã«æããããšã«ããŸããããã«ããGCNãããŒã¿ããåŠç¿ããããšãå¯èœã«ãªããŸãïŒè𳿳šïŒèšãæããã°ãGCNã®åŠç¿ãšã¯ãã®éã¿è¡åã®èŠçŽ ãæé©åããããšã§ãïŒããã®æäœã¯æ¬¡ã®ããã«èšè¿°ãããŸãïŒ
\(i\) ã¯çç®ããŠããããŒãã \(j\) ã¯ãã®è¿åã€ã³ããã¯ã¹, \(k\) ã¯ããŒãã®å ¥åç¹åŸŽã \(l\) ã¯ããŒãã®åºåç¹åŸŽã \(d_i\) ã¯ããŒãã®æ¬¡æ°ïŒæ¬¡æ°ã§å²ãããšã§åãªãå ç®ã§ã¯ãªãå¹³åã«ãªããŸãïŒã \(e_{ij}\) ã¯ãå šãŠã®éè¿åããŒãã \(v_{jk}\) ãŒãã«ãªãããè¿åãšéè¿åããŒããåé¢ããé ã \(\sigma\) ã¯æŽ»æ§å颿°ã \(w_{lk}\) ã¯åŠç¿å¯èœãªéã¿ã§ãã ãã®åŒã¯ãšãŠãé·ãããã«èŠããŸãããå®éã«ãã£ãŠããããšã¯ãè¿åå士ã®å¹³åã«åŠç¿å¯èœãªéã¿è¡åã远å ããã ãã§ãããã®åŒã®ããããæ¡åŒµãšããŠãåããŒãã®è¿åãšããŠèªåèªèº«ãå ããå ŽåããããŸããããã¯ããŒãã®åºåç¹åŸŽ \(v_{il}\) ãå ¥åç¹åŸŽ \(v_{ik}\) ã«äŸåããããã«ããããã§ããããããæã ã¯ãã®ããã«äžã®åŒãä¿®æ£ããå¿ èŠã¯ãããŸããããã£ãšã·ã³ãã«ãªããæ¹ãšããŠãããŒã¿ã®ååŠçã§æçè¡åãå ç®ããè¿æ¥è¡åã®å¯Ÿè§æåã \(0\) ã§ã¯ãªã\(1\) ã«ããŠããã°ããã®ã§ãã
GCNã«ã€ããŠã®çè§£ãæ·±ããããšã¯ãä»ã®çš®é¡ã®GNNãçè§£ããããã«éèŠã§ããããã§ã¯2ã€ã®ãã€ã³ããæŒãããŠãã ããããŸãGCNã¬ã€ã€ãŒã¯ãããŒããšãã®è¿åã®éã§âéä¿¡âããæ¹æ³ãšèŠãããšãã§ããŸããããŒã \(i\) ã«ã€ããŠã®åºåã¯ããã®ããé£ã®ããŒãã«ã®ã¿äŸåããããšã«ãªããŸãããååŠã®å Žåãããã§ã¯äžååã§ããããé æ¹ã®ããŒãã®æ å ±ãåã蟌ãããã«ãæã ã¯è€æ°ã®GCNã¬ã€ã€ãŒãéããããšãã§ããŸãããã2ã€ã®GCNã¬ã€ã€ãŒãããã°ãããŒã \(i\) ã®åºåã¯ããã®é£ã®é£ã®ããŒãã®æ å ±ãå«ãããšã«ãªããŸãã GCNã§çè§£ãã¹ãããäžã€ã®ç¹ã¯ãããŒãç¹åŸŽãå¹³ååããã¹ãããã2ã€ã®ç®çãéããŠããããšã§ãïŒi)è¿åããŒãã®é åºãç¡èŠããããšã§é åºã«å¯ŸããŠç䟡ãšãªããii)ããŒãç¹åŸŽã®ããŒã¿ã®ã¹ã±ãŒã«å€åãé²ããåã«ããŒãç¹åŸŽã®åãåã£ãå Žåã(i)ã¯å®çŸããŸãããåã¬ã€ã€ãŒãéãããšã«ããŒãç¹åŸŽã®ããŒã¿ã®å€ã倧ãããªã£ãŠããŸããŸãããã¡ãããç¹åŸŽã®ã¹ã±ãŒã«ãæããããã«åGCNã¬ã€ã€ãŒã®åŸã§ãããå¹³ååïŒbatch normalizationïŒã¬ã€ã€ãŒãéããšãã察åŠããããŸãããå¹³ååã¯ããã·ã³ãã«ã§ãã
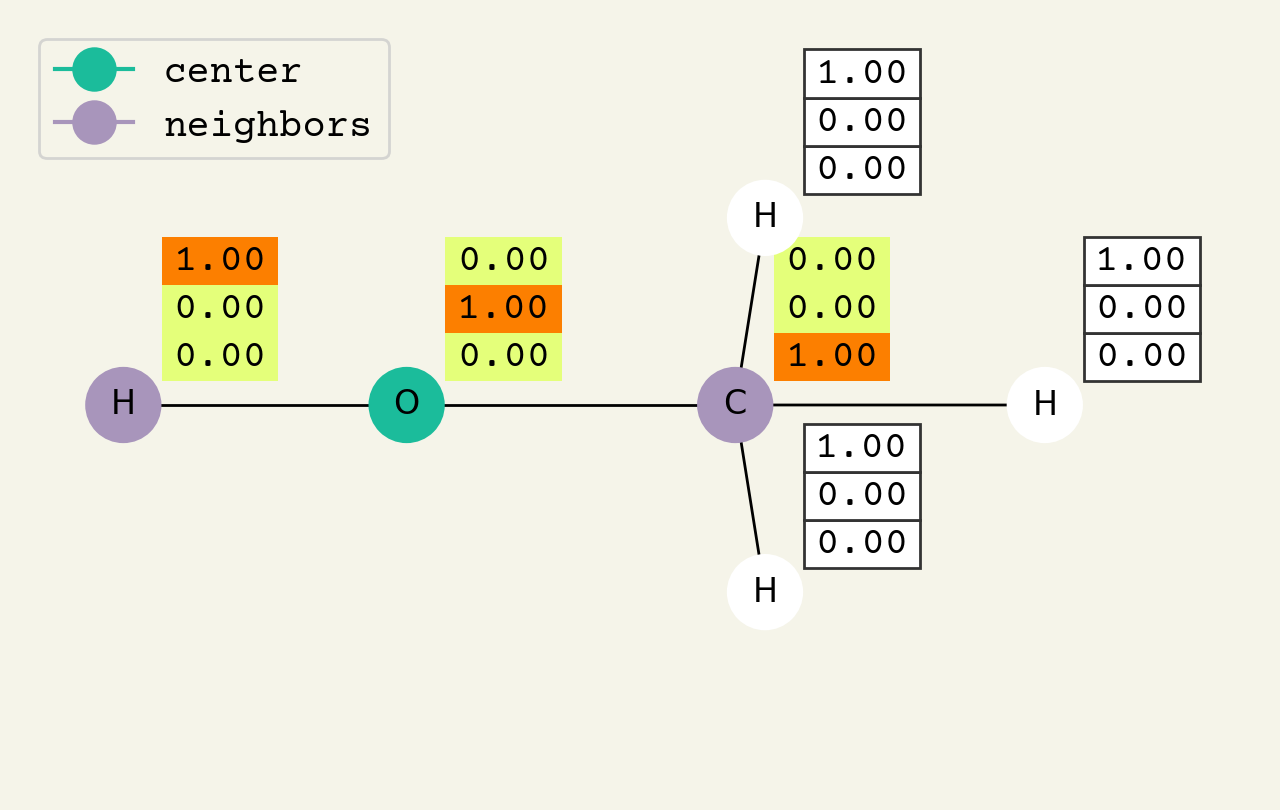
Fig. 8.2 ã°ã©ãç³ã¿èŸŒã¿ã¬ã€ã€ãŒã®äžéã¹ãããã3次å
ãã¯ãã«ã¯ããŒãç¹åŸŽã§ãããåæå€ã¯æ°ŽçŽ ã衚ãone-hotãã¯ãã« [1.00, 0.00, 0.00]
ã§ãããã®äžå¿ããŒãã¯ã飿¥ããããŒãã®ç¹åŸŽãå¹³åããããšã§æŽæ°ãããŠãããŸãã¶
GCNã¬ã€ã€ãŒãçè§£ããããããããã Fig. 8.2 ãèŠãŠãã ãããããã¯GCNã¬ã€ã€ãŒã®äžéã¹ãããã衚ããŠããŸããåããŒãã®ç¹åŸŽã¯ãããã§ã¯one-hot encodingããããã¯ãã«ãšããŠè¡šçŸãããŠããŸããFig. 8.3 ã®ã¢ãã¡ãŒã·ã§ã³ã¯ãè¿åç¹åŸŽã«ã€ããŠã®å¹³ååããã»ã¹ã衚ããŠããŸãããã®ã¢ãã¡ãŒã·ã§ã³ã§ã¯ãããããããããããã«åŠç¿å¯èœãªéã¿ãšæŽ»æ§å颿°ã¯èšè¿°ãããŠããŸããããã®ã¢ãã¡ãŒã·ã§ã³ã¯2å±€ç®ã®GCNã¬ã€ã€ãŒã§ãç¹°ãè¿ãããããšã«æ³šæããŠãã ãããååäžã«é žçŽ ååãå«ãŸãããšããâæ å ±âãã2å±€ç®ã§ã¯ãããŠåååã«äŒæ¬ãããæ§åãããèŠãŠãã ãããå šãŠã®GNNã¯äŒŒããããªã¢ãããŒãã§åäœããã®ã§ããã®ã¢ãã¡ãŒã·ã§ã³ã®å 容ã¯ãšãŠã倧åã§ãããã²ãããçè§£ããŠãã ããã
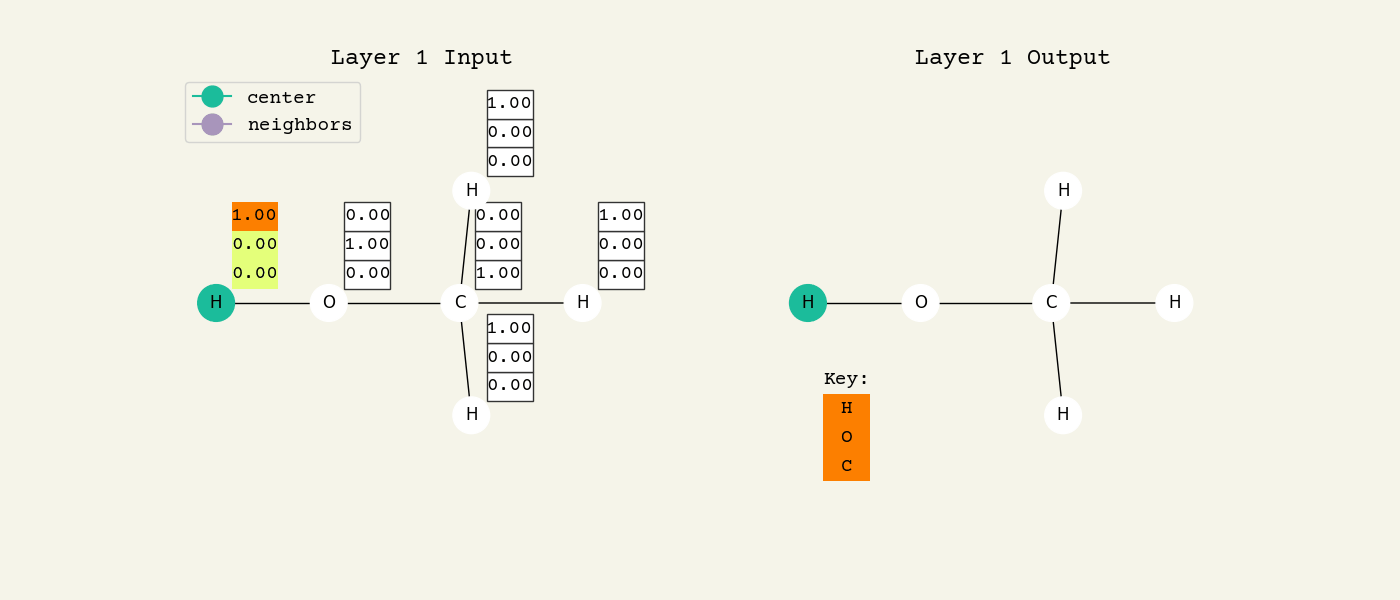
Fig. 8.3 ã°ã©ãç³ã¿èŸŒã¿å±€ã®åäœã®ã¢ãã¡ãŒã·ã§ã³ãå·Šãå ¥åãå³ãåºåããŒãã®ç¹åŸŽã§ãã2ã€ã®å±€ã衚瀺ãããŠããããšã«æ³šæããŠãã ããïŒã¿ã€ãã«ãå€ããããšã«æ³šæããŠèŠãŠãã ããïŒãã¢ãã¡ãŒã·ã§ã³ãé²ãã«ã€ããŠãè¿åããŒãã®å¹³ååã«ãã£ãŠãååã«ã€ããŠã®æ å ±ãã©ã®ããã«ååå ãäŒæããŠãããããããããšã§ããããã€ãŸããé žçŽ ã¯åãªãé žçŽ ãããCãšHã«çµåããé žçŽ ãHãšCH3ã«çµåããé žçŽ ãžãšå€åããŠããã®ã§ããå³äžã®è²ã¯æ°å€ã«å¯Ÿå¿ããŠããŸãã¶
8.4.1. GCNã®å®è£ ¶
ããã§ã¯ãGCNã®ãã³ãœã«å®è£
ãäœããŸããããããã§ã¯äžæŠã掻æ§å颿°ããã³åŠç¿å¯èœãªéã¿ã«ã€ããŠã¯çç¥ããŸãã
ãŸãæåã«ãã©ã³ã¯2ã®é£æ¥è¡åãèšç®ããå¿
èŠããããŸããäžã® smiles2graph
ã³ãŒãã¯ãç¹åŸŽãã¯ãã«ãçšããŠé£æ¥è¡åãèšç®ããŸãããã®èšç®ã¯ç°¡åã§ããåæã«æçè¡åãå ããããšã«ããŸãïŒè𳿳šïŒãã®æçè¡åãå ç®ããæäœã¯ãäžã§è¿°ã¹ãããã«èªåèªèº«ã飿¥ããŒããšããŠåãæ±ãããã®å·¥å€«ã§ãïŒã
nodes, adj = smiles2graph("CO")
adj_mat = np.sum(adj, axis=-1) + np.eye(adj.shape[0])
adj_mat
array([[1., 1., 1., 1., 1., 0.],
[1., 1., 0., 0., 0., 1.],
[1., 0., 1., 0., 0., 0.],
[1., 0., 0., 1., 0., 0.],
[1., 0., 0., 0., 1., 0.],
[0., 1., 0., 0., 0., 1.]])
åããŒãã®æ¬¡æ°ãèšç®ããããã«ããŸãå¥ãªçž®çŽæäœãè¡ããŸãïŒ
degree = np.sum(adj_mat, axis=-1)
degree
array([5., 3., 2., 2., 2., 2.])
ããã§ããŒãã®æŽæ°æäœã®æºåãã§ããŸãããã¢ã€ã³ã·ã¥ã¿ã€ã³ã®çž®çŽèšæ³ã䜿ã£ãп޿°æäœã衚çŸãããšæ¬¡ã®ããã«ãªããŸã
print(nodes[0])
# note to divide by degree, make the input 1 / degree
new_nodes = np.einsum("i,ij,jk->ik", 1 / degree, adj_mat, nodes)
print(new_nodes[0])
[1. 0. 0.]
[0.2 0.2 0.6]
ãããKerasã®LayerãšããŠå®è£
ããã«ã¯ãäžèšã®ã³ãŒããæ°ããLayerã®ãµãã¯ã©ã¹ãšããŠèšè¿°ããå¿
èŠããããŸããä»åã®ã³ãŒãã¯æ¯èŒçç°¡åã§ãããKerasã®é¢æ°åãšLayerã¯ã©ã¹ã«ã€ããŠããã®ãã¥ãŒããªã¢ã«ãèªãã§åŠã¶ããšãæšå¥šããŸããäž»ãªå€æŽç¹ã¯ãåŠç¿å¯èœãªãã©ã¡ãŒã¿ self.w
ãäœã£ãŠ tf.einsum
ã®äžã§çšããããšã掻æ§å颿° self.activation
ãçšããããšããããŠæ°ããããŒãç¹åŸŽãšé£æ¥è¡åãåºåããããšã®3ç¹ã§ãã飿¥è¡åãåºåããçç±ã¯ã飿¥è¡åãæ¯åæž¡ãããšãªããè€æ°ã®GCNã¬ã€ã€ãŒãã¹ã¿ãã¯ã§ããããã«ããããã§ãã
class GCNLayer(tf.keras.layers.Layer):
"""Implementation of GCN as layer"""
def __init__(self, activation=None, **kwargs):
# constructor, which just calls super constructor
# and turns requested activation into a callable function
super(GCNLayer, self).__init__(**kwargs)
self.activation = tf.keras.activations.get(activation)
def build(self, input_shape):
# create trainable weights
node_shape, adj_shape = input_shape
self.w = self.add_weight(shape=(node_shape[2], node_shape[2]), name="w")
def call(self, inputs):
# split input into nodes, adj
nodes, adj = inputs
# compute degree
degree = tf.reduce_sum(adj, axis=-1)
# GCN equation
new_nodes = tf.einsum("bi,bij,bjk,kl->bil", 1 / degree, adj, nodes, self.w)
out = self.activation(new_nodes)
return out, adj
äžèšã®ã³ãŒãã®å€§åã¯Keras/TFã«åºæã®ãã®ã§ã倿°ãé©åãªå Žæã«é 眮ããŠããŸããããã§éèŠãªã®ã¯2è¡ã ãã§ãã1ã€ãã¯ã飿¥è¡åã®åã«ã€ããŠåèšããããšã§ã°ã©ãã®æ¬¡æ°ãèšç®ããæäœã§ãïŒ
degree = tf.reduce_sum(adj, axis=-1)
2ã€ãã®éèŠãªè¡ã¯ãGCNæ¹çšåŒ (8.3) ãèšç®ããéšåã§ãïŒããã§ã¯æŽ»æ§åã¯çç¥ããŠããŸãïŒïŒ
new_nodes = tf.einsum("bi,bij,bjk,kl->bil", 1 / degree, adj, nodes, self.w)
ããã§ãããŸå®è£ ããGCNã¬ã€ã€ãŒã詊ãããšãã§ããããã«ãªããŸããïŒ
gcnlayer = GCNLayer("relu")
# we insert a batch axis here
gcnlayer((nodes[np.newaxis, ...], adj_mat[np.newaxis, ...]))
(<tf.Tensor: shape=(1, 6, 3), dtype=float32, numpy=
array([[[0. , 0.46567526, 0.07535715],
[0. , 0.12714943, 0.05325063],
[0.01475453, 0.295794 , 0.39316285],
[0.01475453, 0.295794 , 0.39316285],
[0.01475453, 0.295794 , 0.39316285],
[0. , 0.38166213, 0. ]]], dtype=float32)>,
<tf.Tensor: shape=(1, 6, 6), dtype=float32, numpy=
array([[[1., 1., 1., 1., 1., 0.],
[1., 1., 0., 0., 0., 1.],
[1., 0., 1., 0., 0., 0.],
[1., 0., 0., 1., 0., 0.],
[1., 0., 0., 0., 1., 0.],
[0., 1., 0., 0., 0., 1.]]], dtype=float32)>)
ããã«ãã (1) æ°ããããŒãç¹åŸŽã(2) 飿¥è¡åãåºåãããŸãããã®ã¬ã€ã€ãŒãç©ã¿éããŠãGCNãè€æ°åé©çšã§ããããšã確èªããŸãããã
x = (nodes[np.newaxis, ...], adj_mat[np.newaxis, ...])
for i in range(2):
x = gcnlayer(x)
print(x)
(<tf.Tensor: shape=(1, 6, 3), dtype=float32, numpy=
array([[[0. , 0.18908624, 0. ],
[0. , 0. , 0. ],
[0. , 0.145219 , 0. ],
[0. , 0.145219 , 0. ],
[0. , 0.145219 , 0. ],
[0. , 0. , 0. ]]], dtype=float32)>, <tf.Tensor: shape=(1, 6, 6), dtype=float32, numpy=
array([[[1., 1., 1., 1., 1., 0.],
[1., 1., 0., 0., 0., 1.],
[1., 0., 1., 0., 0., 0.],
[1., 0., 0., 1., 0., 0.],
[1., 0., 0., 0., 1., 0.],
[0., 1., 0., 0., 0., 1.]]], dtype=float32)>)
ããŸããããŸããïŒãããããªããŒãã®å€ãããã®ã§ããããïŒããã¯ãããããåºåã«è² ã®å€ãå«ãŸããŠããããããReLU掻æ§åãéããéã«0ã«ãªã£ãããã§ããããããã¯ã¢ãã«ã®èšç·Žãäžååãªããã«èµ·ããŠãããšèããããèšç·Žãéããããšã§è§£æ±ºããã§ããã
8.5. äŸïŒæº¶è§£åºŠäºæž¬Â¶
次ã«ãGCNã«ããæº¶è§£åºŠã®äºæž¬ã«ã€ããŠèª¬æããŸãã以åã«ãååããŒã¿ã»ããã«å«ãŸããŠããç¹åŸŽéã䜿ã£ãŠäºæž¬ã¢ãã«ãçµãã ããšãæãåºããŠãã ãããããŸæã ã¯GCNã䜿ããããã«ãªã£ãã®ã§ãç¹åŸŽéã«é ãæ©ãŸãããšãªããååæ§é ãçŽæ¥ãã¥ãŒã©ã«ãããã¯ãŒã¯ã«å ¥åã§ããããã«ãªããŸãããGCNã¬ã€ã€ãŒã¯åããŒãã«ã€ããŠã®ç¹åŸŽãåºåããŸãããæº¶è§£åºŠãäºæž¬ããããã«ã¯ãã°ã©ãå šäœã«ã€ããŠã®ç¹åŸŽãåŸãå¿ èŠããããŸãããã®ããã»ã¹ãããã«æŽç·Žããæ¹æ³ã«ã€ããŠã¯åŸã§èª¬æããŸãããããã§ã¯ãGCNã¬ã€ã€ãŒåŸã®ãã¹ãŠã®ããŒãç¹åŸŽã®å¹³åã䜿ãããšã«ããŸããããã«ããåçŽãã€é åºäžå€ã«ãããŒãç¹åŸŽãã°ã©ãç¹åŸŽã«å€æããããšãã§ããŸãããã®å®è£ ã¯æ¬¡ã®ãšããã§ãïŒ
class GRLayer(tf.keras.layers.Layer):
"""A GNN layer that computes average over all node features"""
def __init__(self, name="GRLayer", **kwargs):
super(GRLayer, self).__init__(name=name, **kwargs)
def call(self, inputs):
nodes, adj = inputs
reduction = tf.reduce_mean(nodes, axis=1)
return reduction
äžã®ã³ãŒãã§éèŠãªç¹ã¯ãããŒãã«ã€ããŠå¹³åããšã£ãŠãã(axis=1
)次ã®éšåã ãã§ãïŒ
reduction = tf.reduce_mean(nodes, axis=1)
ãã®æº¶è§£åºŠäºæž¬åšã宿ããããããããã€ãã®å šçµåå±€ã远å ããŠãååž°ãè¡ããããšã確èªããŸããããååž°ã®å Žåã¯æçµå±€ã®åºåããã®ãŸãŸäºæž¬çµæãšãªããããæçµå±€ã«ã¯æŽ»æ§åãé©çšããªãããšã«æ³šæããŠãã ããããã®ã¢ãã«ã¯ Keras functional API ã䜿ã£ãŠå®è£ ãããŠããŸãã
ninput = tf.keras.Input(
(
None,
100,
)
)
ainput = tf.keras.Input(
(
None,
None,
)
)
# GCN block
x = GCNLayer("relu")([ninput, ainput])
x = GCNLayer("relu")(x)
x = GCNLayer("relu")(x)
x = GCNLayer("relu")(x)
# reduce to graph features
x = GRLayer()(x)
# standard layers (the readout)
x = tf.keras.layers.Dense(16, "tanh")(x)
x = tf.keras.layers.Dense(1)(x)
model = tf.keras.Model(inputs=(ninput, ainput), outputs=x)
ãã®100ã¯ã©ãããæ¥ãã®ã§ããããïŒãã®çãã¯ããŒã¿ã»ããã«å«ãŸããå
çŽ ã®æ°ã«ãããŸãããã®ããŒã¿ã»ããã¯å€æ°ã®å
çŽ ãå«ãããã以å䜿ã£ããµã€ãº3ã®one-hot encodingã§ã¯ãå
šãŠã®å
çŽ ã衚çŸã§ããŸãããååã¯C, H, Oã®å
çŽ ãã衚çŸã§ããã°ååã§ããããä»åã¯ãã倿°ã®å
çŽ ãæ±ãå¿
èŠããããŸãããã®ãããone-hot encodingã®ãµã€ãºã100ã«å¢ããããšã«ããŸãããããã§æå€§100çš®é¡ã®å
çŽ ã衚çŸã§ããŸãããã®æ¡åŒµã®ããã«ãã¢ãã«ã ãã§ãªãsmiles2graph
颿°ãæŽæ°ããããšã«ããŸãããã
def gen_smiles2graph(sml):
"""Argument for the RD2NX function should be a valid SMILES sequence
returns: the graph
"""
m = rdkit.Chem.MolFromSmiles(sml)
m = rdkit.Chem.AddHs(m)
order_string = {
rdkit.Chem.rdchem.BondType.SINGLE: 1,
rdkit.Chem.rdchem.BondType.DOUBLE: 2,
rdkit.Chem.rdchem.BondType.TRIPLE: 3,
rdkit.Chem.rdchem.BondType.AROMATIC: 4,
}
N = len(list(m.GetAtoms()))
nodes = np.zeros((N, 100))
for i in m.GetAtoms():
nodes[i.GetIdx(), i.GetAtomicNum()] = 1
adj = np.zeros((N, N))
for j in m.GetBonds():
u = min(j.GetBeginAtomIdx(), j.GetEndAtomIdx())
v = max(j.GetBeginAtomIdx(), j.GetEndAtomIdx())
order = j.GetBondType()
if order in order_string:
order = order_string[order]
else:
raise Warning("Ignoring bond order" + order)
adj[u, v] = 1
adj[v, u] = 1
adj += np.eye(N)
return nodes, adj
nodes, adj = gen_smiles2graph("CO")
model((nodes[np.newaxis], adj_mat[np.newaxis]))
<tf.Tensor: shape=(1, 1), dtype=float32, numpy=array([[0.0107595]], dtype=float32)>
ãã®ã¢ãã«ã¯1ã€ã®æ°å€ïŒã¹ã«ã©ãŒïŒãåºåããŸãã
ããŠãåŠç¿å¯èœãªããŒã¿ã»ãããåŸãããã«ãããã€ãäœæ¥ãå¿
èŠã§ãããã®ããŒã¿ã»ããã¯å°ãè€éã§ãç¹åŸŽã¯ãã³ãœã«(\(mathbf{V}, \mathbf{E}\))ã®ã¿ãã«ãªã®ã§ãããŒã¿ã»ããã¯æ¬¡ã®ãããªã¿ãã«ã®ã¿ãã«ã«ãªããŸãïŒ \(\left((\mathbf{V}, \mathbf{E}), y\right)\) generatorã¯Pythonã®é¢æ°ã§ãå€ãç¹°ãè¿ãè¿ãããšãã§ããŸããããã§ã¯ãåŠç¿ããŒã¿ã1ã€ãã€åãåºãããã«generatorã䜿ããŸããç¶ããŠãããã from_generator
tf.data.Dataset
ã³ã³ã¹ãã©ã¯ã¿ã«æž¡ããŸãããã®ã³ã³ã¹ãã©ã¯ã¿ã§ã¯ãå
¥åããŒã¿ã®shapeãæç€ºçã«æå®ããå¿
èŠããããŸãã
def example():
for i in range(len(soldata)):
graph = gen_smiles2graph(soldata.SMILES[i])
sol = soldata.Solubility[i]
yield graph, sol
data = tf.data.Dataset.from_generator(
example,
output_types=((tf.float32, tf.float32), tf.float32),
output_shapes=(
(tf.TensorShape([None, 100]), tf.TensorShape([None, None])),
tf.TensorShape([]),
),
)
ãããŸã§æ¥ããããå°ãã§ããããã§ããã€ãã®ããã«ããŒã¿ã»ãããtrain/val/testã«åå²ã§ããŸãã
test_data = data.take(200)
val_data = data.skip(200).take(200)
train_data = data.skip(400)
ãããŠãããããã¢ãã«ã®èšç·Žã§ã
model.compile("adam", loss="mean_squared_error")
result = model.fit(train_data.batch(1), validation_data=val_data.batch(1), epochs=10)
plt.plot(result.history["loss"], label="training")
plt.plot(result.history["val_loss"], label="validation")
plt.legend()
plt.xlabel("Epoch")
plt.ylabel("Loss")
plt.show()
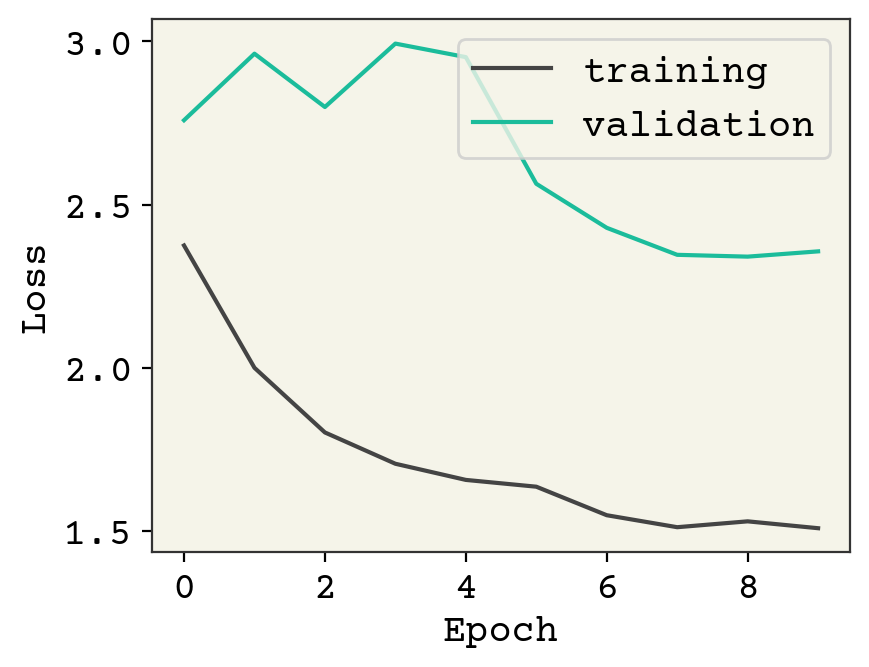
ãã®ã¢ãã«ã¯æããã«ã¢ã³ããŒãã£ããã§ããèããããçç±ã®äžã€ã¯ããããµã€ãºã1ã§ããããšã§ãããã®ã¢ãã«ã§ã¯ãåååæ°ãå¯å€ã«ããå¯äœçšãšããŠããããµã€ãºã1ãã倧ããã§ããªãå¶çŽããããŸããããå°ã詳ãã説æãããšãä»»æã®ããããµã€ãºãå ¥åã§ããããã«ãããšãåååæ°ãšããããµã€ãºã®2ã€ãäžå®ãšãªããŸããKeras/tensorflowã¯å ¥åããŒã¿ã®shapeã«æªç¥ã®æ¬¡å ã2ã€ä»¥äžããå ŽåããŒã¿ãåŠçã§ããªããããããããµã€ãºã1ã«åºå®ããããšã§å¯ŸåŠããŠããŸããããã§ã¯æ±ããŸãããããã®åé¡ãåé¿ããŠããããµã€ãºã1ãã倧ããããããã®æšæºçããªãã¯ã¯ãè€æ°ã®ååãïŒååéã®çµåããªãïŒ1ã€ã®ã°ã©ãã«ãŸãšããŠããŸãããšã§ããããã«ããããŒã¿ã®æ¬¡å ã¯ãã®ãŸãŸã«ãååããããåŠçããããšãã§ããŸãã
ããã§ã¯ããªãã£ããããã§äºæž¬ç²ŸåºŠã確èªããŠã¿ãŸãããã
yhat = model.predict(test_data.batch(1), verbose=0)[:, 0]
test_y = [y for x, y in test_data]
plt.figure()
plt.plot(test_y, test_y, "-")
plt.plot(test_y, yhat, ".")
plt.text(
min(test_y) + 1,
max(test_y) - 2,
f"correlation = {np.corrcoef(test_y, yhat)[0,1]:.3f}",
)
plt.text(
min(test_y) + 1,
max(test_y) - 3,
f"loss = {np.sqrt(np.mean((test_y - yhat)**2)):.3f}",
)
plt.title("Testing Data")
plt.show()
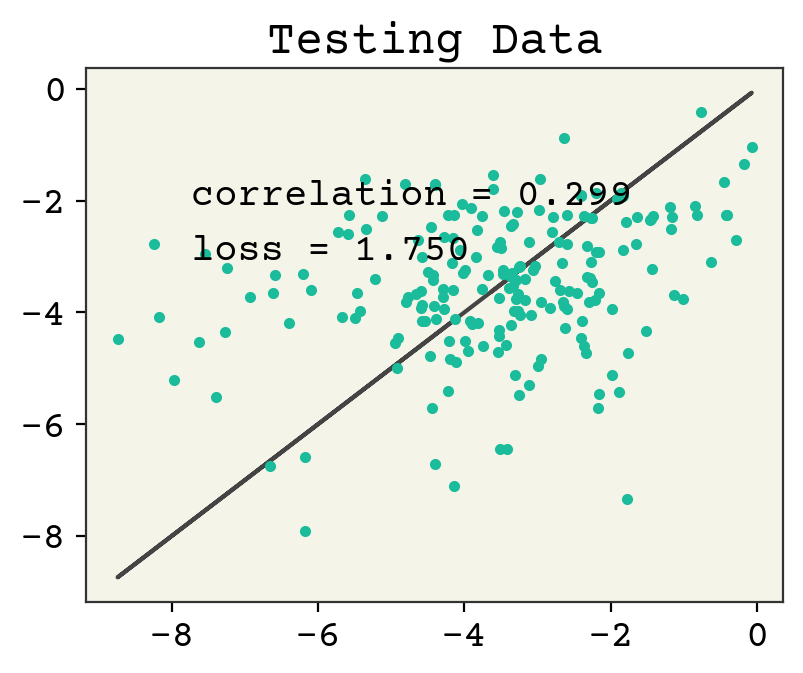
8.6. Message PassingãšGCN¶
ããåºãæå³ã§GCNã¬ã€ã€ãŒãæãããšãGCNã¬ã€ã€ãŒã¯âmessage-passingâã¬ã€ã€ãŒã®äžã€ãšèšããŸããGCNã§ã¯ããŸãè¿åããŒããããã£ãŠããã¡ãã»ãŒãžãåŠçããŸãïŒ
ããã§ \(v_{{s_i}j}\) 㯠ããŒã \(i\) ã® \(j\) çªç®ã®è¿åã§ãã \(s_i\) 㯠\(i\) ã«å¯Ÿããã»ã³ããŒïŒéä¿¡è ïŒã§ãã ããã¯GCNãã©ã®ããã«ã¡ãã»ãŒãžãèšç®ããã瀺ãããã®ã§ããããã£ãŠããããšã¯åçŽã§ãåè¿åããŒãã®ç¹åŸŽã«éã¿è¡åããããŠããã ãã§ããããŒã \(i\) ã«åããã¡ãã»ãŒãž \(\vec{e}_{{s_i}j}\) ãåŸãåŸããããã®ã¡ãã»ãŒãžãããŒãã®é çªã«å¯ŸããŠäžå€ãªé¢æ°ãçšããŠéçŽããŸãïŒ
äžã§æ±ã£ãããã«ãGCNã§ã¯ãã®éçŽã¯åãªãå¹³åã§ãããä»»æã®ïŒäŸãã°åŠç¿å¯èœãªïŒé åºäžå€ã®é¢æ°ã䜿ãããšãã§ããŸãïŒ
\(v^{'}\) ã¯æ°ããããŒãç¹åŸŽã瀺ããŠããŸããããã¯åçŽã«ãéçŽãããåŸã§æŽ»æ§å颿°ãé©çšããã¡ãã»ãŒãžã§ãããã®ããã«æžãåºãããšã§ããããã®æé ã«ãããã§ãå°ããªå€æŽãå ããããããšã«æ°ã¥ããã®ã§ã¯ãªãã§ãããããGilmerãã«ããéèŠãªè«æ [GSR+17] ã§ã¯ãããã€ãã®éžæè¢ãæ€èšãããã®ã¡ãã»ãŒãžããã·ã³ã°ã¬ã€ã€ãŒã®åºæ¬çãªã¢ã€ãã£ã¢ããéåååŠã«åºã¥ããŠååãšãã«ã®ãŒãäºæž¬ããã¿ã¹ã¯ã§ããŸãããããšãè¿°ã¹ãããŠããŸããGCNåŒã«å€æŽãå ããäŸãšããŠã¯ãè¿åã¡ãã»ãŒãžã®èšç®ã«ãããŠãšããžç¹åŸŽãå«ããããåã« \(\sigma\) ã§åããšã代ããã«å šçµåå±€ã䜿ããšãã£ã詊ã¿ãæããããŸãã ããããããGCNã¯ãã¡ãã»ãŒãžããã·ã³ã°ã°ã©ããã¥ãŒã©ã«ãããã¯ãŒã¯ïŒMPNNãšç¥ãããããšããããŸãïŒã®äžçš®ãšèããããšãã§ããŸãã
8.7. Gated Graph Neural Network¶
ã¡ãã»ãŒãžããã·ã³ã°ã¬ã€ã€ãŒã®æãæåãªäºçš®ã®äžã€ã¯ã gated graph neural network (GGN) [LTBZ15] ã§ããããã¯æåŸã®åŒã®ããŒãæŽæ°ã次ã§çœ®ãæãããã®ã§ãïŒ
\(\textrm{GRU}(\cdot, \cdot)\) ã¯ã²ãŒãååž°ãŠãããïŒgated recurrent unitïŒ[CGCB14] ã§ãã GRU ã¯ãã€ããªïŒå ¥ååŒæ°ã2ã€æã€ïŒãã¥ãŒã©ã«ãããã¯ãŒã¯ã§ãå žåçã«ã¯ç³»åããŒã¿ã®ã¢ããªã³ã°ã«äœ¿çšãããŸããGCNãšæ¯èŒããŠãGGNã®è峿·±ãç¹åŸŽã¯ãïŒGRUããã®ïŒããŒãæŽæ°ã«ãããŠåŠç¿å¯èœãªãã©ã¡ãŒã¿ãæã€ããšã§ãããæè»æ§ãåããã¢ãã«ãšãªã£ãŠããããšã§ããGGNã§ã¯ãGRUã®ãã©ã¡ãŒã¿ã¯åå±€ã§å ±æãããŸãïŒGRUã䜿ã£ãŠç³»åããŒã¿ãã¢ããªã³ã°ããæ¹æ³ãšåãã§ãïŒããã©ã¡ãŒã¿å ±æã«ããã¡ãªããã¯ãåŠç¿ãã¹ããã©ã¡ãŒã¿ãå¢ããããšãªãGGNã®å±€ãç¡éã«ç©ã¿éããããããšã§ãïŒå局㧠\(\mathbf{W}\) ãæããããšãåæã§ãïŒããã®ãããGGNã¯å€§ããªã¿ã³ãã¯è³ªã倧ããªãŠãããã»ã«ããã€çµæ¶æ§é ã®ãããªã倧ããªã°ã©ãã«é©ããŠããŸãïŒè𳿳šïŒGGNã¬ã€ã€ãŒãäœå±€ãã¹ã¿ãã¯ããããšã§ãããé ãã®ããŒãããã®æ å ±ãåã蟌ãããšãã§ããããã§ãïŒã
8.8. Pooling¶
ã¡ãã»ãŒãžããã·ã³ã°ã®èгç¹ãããã³äžè¬ã«GNNSã§ã¯ãè¿åããã®ã¡ãã»ãŒãžãçµåããæ¹æ³ãéèŠãªã¹ããããšãªããŸãããã®ã¹ãããã¯ç³ã¿èŸŒã¿ãã¥ãŒã©ã«ãããã¯ãŒã¯ã§äœ¿ãããããŒãªã³ã°å±€ã«äŒŒãŠãããããããŒãªã³ã°ãšåŒã°ããããšããããŸããç³ã¿èŸŒã¿ãã¥ãŒã©ã«ãããã¯ãŒã¯ã®ããŒãªã³ã°ãšåãããã«ããã®ããã«äœ¿çšã§ããçž®çŽæäœã¯è€æ°ãããŸããäžè¬çã«ãGNNã®ããŒãªã³ã°ã«ã¯ã¡ãã»ãŒãžã®åèšãŸãã¯å¹³åã䜿ãããŸãããã°ã©ãååãããã¯ãŒã¯ïŒGraph Isomorphism NetworksïŒ [XHLJ18] ã®ããã«éåžžã«æŽç·Žãããæäœãçšããããšãã§ããŸãããã®ä»ã«ããäŸãã°æ³šæïŒattentionïŒã®ç« ã§ã¯èªå·±æ³šæïŒself-attentionïŒã䜿ãäŸãæ±ããŸããããããã®æäœãããŒãªã³ã°ã«äœ¿ããŸããããŒãªã³ã°ã®ã¹ããããè²ã ãšå·¥å€«ããããªãããšããããŸãããããŒãªã³ã°æäœã®éžæã¯ã¢ãã«ã®æ§èœã«ãšã£ãŠããã»ã©éèŠã§ã¯ãªãããšãçµéšçã«åãã£ãŠããŸã [LDLio19, MSK20]ãããŒãªã³ã°ã®éèŠãªç¹æ§ã¯é åºäžå€æ§ã§ãéçŽæäœã¯ããŒãïŒããŒãªã³ã°ã®å Žåã¯ãšããžïŒã®é åºã«äŸåããªãæ§è³ªãåããããšãæãŸããŸããGrattarolaã [GZBA21]ããããŒãªã³ã°ææ³ã«é¢ããæè¿ã®ã¬ãã¥ãŒãåºçããŠããŸãã
Daigavaneãã®è«æ [DRA21] ã§ã¯ãæ§ã ãªããŒãªã³ã°æŠç¥ã®æ¯èŒãšæŠèŠãèŠèŠçã«ç޹ä»ãããŠããŸãã
8.9. Readout Function¶
GNNã®åºåã¯ã°ã©ãã§ãïŒããèšèšãããŠããã®ã§åœããåã§ããïŒãããããäºæž¬ãããã©ãã«ãã°ã©ãã§ããããšã¯çšã§ãäžè¬çã«ã¯ã©ãã«ã¯åããŒããŸãã¯ã°ã©ãå šäœã«å¯ŸããŠä»äžãããŠããŸããããŒãã©ãã«ã®äŸã¯ååã®éšåé»è·ãã°ã©ãã©ãã«ã®äŸã¯ååã®ãšãã«ã®ãŒã§ããGNNããåºåãããã°ã©ãããäºæž¬ã¿ãŒã²ããã§ããããŒãã©ãã«ãã°ã©ãã©ãã«ã«å€æããããã»ã¹ã èªã¿åºã ïŒreadoutïŒ ãšåŒã³ãŸããããŒãã©ãã«ãäºæž¬ããå Žåã§ããã°ãåçŽã«ãšããžç¹åŸŽãæšãŠãŠãGNNããåºåãããããŒãç¹åŸŽãã¯ãã«ãäºæž¬çµæãšããŠæ±ãããšãã§ããŸãããã®å Žåãåºåå±€ã®åã«ããã€ãã®å šçµåå±€ãæãããšãå€ãã§ãããã
ååã®ãšãã«ã®ãŒãå®å¹é»è·ã®ãããªã°ã©ãã¬ãã«ã®ã©ãã«ãäºæž¬ããå ŽåãããŒã/ãšããžç¹åŸŽãã°ã©ãã©ãã«ã«å€æããããã»ã¹ã«æ³šæãå¿ èŠã§ããææã®shapeã®ã°ã©ãã©ãã«ãåŸãããã«ãåçŽã«ããŒãç¹åŸŽãå šçµåå±€ã«å ¥åããå Žåãé åºç䟡æ§ã倱ãããŠããŸããŸãïŒåºåã¯ããŒãã©ãã«ã§ã¯ãªãã°ã©ãã©ãã«ãªã®ã§ãå³å¯ã«ã¯ãé åºç䟡æ§ã§ã¯ãªãé åºäžå€æ§ã§ãïŒã溶解床ã®äŸã§çšããèªã¿åºãã¯ãããŒãç¹åŸŽéã«å¯ŸããŠçž®çŽæäœãè¡ãããšã§ããããã®åŸã§ã°ã©ãç¹åŸŽãå šçµåå±€ã«å ¥åããŠäºæž¬çµæãåŸãŸãããå®ã¯ããããã°ã©ãç¹åŸŽã®èªã¿åºããè¡ãå¯äžã®æ¹æ³ã§ããããšã瀺ãããŠããŸã [ZKR+17] ãããªãã¡ãã°ã©ãç¹åŸŽãåŸãããã«ããŒãç¹åŸŽã®çž®çŽæäœãè¡ãããã®ã°ã©ãç¹åŸŽãå šçµåå±€ã«å ¥åããããšã§äºæž¬çµæã§ããã°ã©ãã©ãã«ãåŸãŸããåããŒãã®ç¹åŸŽéã«å¯ŸããŠããããå šçµåå±€ãéãæäœãã§ããŸãããããŒãç¹åŸŽãžã®å šçµåå±€ã®é©çšã¯GNNå éšã§æ¢ã«è¡ãããŠããã®ã§ãããŸããå§ãããŸããããã®ã°ã©ãç¹åŸŽã®èªã¿åºãã¯DeepSetsãšåŒã°ããããšããããŸããããã¯ãç¹åŸŽãéåïŒè𳿳šïŒé åºãæãããåæ°ãäžå®ïŒãšããŠäžããããå Žåã®ããã«èšèšããããé åºäžå€ãªã¢ãŒããã¯ãã£ã§ããDeepSets [ZKR+17] ãšåã圢ã§ããããã§ãã
ããŒãªã³ã°ãèªã¿åºããé åºäžå€ã®é¢æ°ã䜿ãããŠããããšã«ãæ°ã¥ãã§ããããããããã£ãŠãDeepSetsã¯ããŒãªã³ã°ã«ãattentionã¯èªã¿åºãã«äœ¿çšããããšãã§ããŸãã
8.9.1. Intensive vs Extensive¶
ååž°ã¿ã¹ã¯ã§ã®èªã¿åºãã«ãããŠèæ ®ãã¹ãéèŠãªç¹ã®1ã€ã¯ãã©ãã«ã intensive ã extensive ãã§ããIntensiveã©ãã«ã¯ãããŒãïŒïŒååïŒã®æ°ã«äŸåããªãå€ãæã€ã©ãã«ã§ããäŸãã°ã屿çãæº¶è§£åºŠãªã©ã¯Intensiveã©ãã«ã§ããIntensiveã©ãã«ã®èªã¿åºãã¯ãäžè¬ã«ããŒãã®æ°ã«äŸåããªãããšãèŠè«ãããŸãããããã£ãŠããã®å Žåã®èªã¿åºãã«ãããçž®çŽæäœãšããŠãå¹³åãæå€§ããšãæäœã¯é©çšå¯èœã§ãããããŒãæ°ã«ããå€ãå€ããããåèšã¯é©ããŸãããå¯Ÿç §çã«ãExtensiveã©ãã«ã§ã¯ãïŒäžè¬çã«ã¯ïŒèªã¿åºãã®çž®çŽæäœã«ã¯ïŒããŒãæ°ãåæ ã§ããããïŒåèšãé©ããŸããextensiveãªååç¹æ§ã®äŸã«ã¯ãçæãšã³ã¿ã«ããŒãæããããŸãã
8.10. Battaglia General Equations¶
ãããŸã§ã§åŠãã ããã«ãGNNã¬ã€ã€ãŒã¯ã¡ãã»ãŒãžããã·ã³ã°ã¬ã€ã€ãŒãšããŠäžè¬åããããšãã§ããŸãããBattagliaã [BHB+18] ã¯ããã«é²ãã§ãã»ãŒãã¹ãŠã®GNNãèšè¿°ã§ããäžè¬çãªæ¹çšåŒã®éåãèæ¡ããŸããã圌ãã¯GNNã¬ã€ã€ãŒã®æ¹çšåŒããã¡ãã»ãŒãžããã·ã³ã°ã¬ã€ã€ãŒã®æ¹çšåŒã«ãããããŒãæŽæ°åŒã®ãããª3ã€ã®æŽæ°æ¹çšåŒãšã3ã€ã®éçŽæ¹çšåŒãšããåèš6ã€ã®åŒã«åè§£ããŸããããããã®åŒã§ã¯ãã°ã©ãç¹åŸŽãã¯ãã«ãšããæ°ããæŠå¿µãå°å ¥ãããŠããŸãããã®ã¢ã€ãã£ã¢ã§ã¯ããããã¯ãŒã¯ã«2ã€ã®éšåïŒGNNãšèªã¿åºãïŒãæããã代ããã«ãã°ã©ãã¬ãã«ã®ç¹åŸŽãåGNNã¬ã€ã€ãŒã§æŽæ°ããã¢ãããŒãããšããŸããã°ã©ãç¹åŸŽãã¯ãã«ã¯ãã°ã©ãå šäœã衚ãç¹åŸŽã®éåã§ããäŸãã°æº¶è§£åºŠãèšç®ããå Žåã¯ãèªã¿åºã颿°ãæã€ä»£ããã«ååå šäœã®ç¹åŸŽãã¯ãã«ãæ§ç¯ãããããæŽæ°ããŠæçµçã«æº¶è§£åºŠãäºæž¬ããæ¹æ³ãæå¹ã ã£ãå¯èœæ§ããããŸãããã®ããã«ãååå šäœã«ã€ããŠå®çŸ©ãããããããçš®é¡ã®éïŒäŸïŒæº¶è§£åºŠããšãã«ã®ãŒïŒã¯ãã°ã©ãã¬ãã«ã®ç¹åŸŽãã¯ãã«ãçšããŠäºæž¬ã§ããã§ãããã
ãããã®åŒã®æåã®ã¹ãããã¯ãšããžã®ç¹åŸŽãã¯ãã«ã®æŽæ°ã§ãããæ°ãã«å°å ¥ãã倿°ã§ãã \(\vec{e}_k\) ã«ã€ããŠã®åŒãšããŠèšè¿°ãããŸãïŒ
\(\vec{e}_k\) ã¯ãšããž \(k\)ãã®ç¹åŸŽãã¯ãã«ã \(\vec{v}_{rk}\) ã¯ãšããž \(k\) ã«ã€ããŠåä¿¡ãããããŒãç¹åŸŽãã¯ãã«ã \(\vec{v}_{sk}\) ã¯ãšããž \(k\) ã«ã€ããŠããŒãç¹åŸŽãã¯ãã«ãéä¿¡ããããŒãã \(\vec{u}\) ã¯ã°ã©ãç¹åŸŽãã¯ãã«ã \(\phi^e\) ã¯GNNã¬ã€ã€ãŒã®å®çŸ©ã«äœ¿ããã3çš®é¡ã®æŽæ°é¢æ°ã®ãã¡ã®äžã€ã§ãããã ããããã§èšã3çš®é¡ã®æŽæ°é¢æ°ãšã¯äžè¬åãã衚çŸã§ãããå¿ ããã3çš®é¡ãå®çŸ©ããå¿ èŠã¯ãããŸãããããã§ã¯ãã®ãã¡ã®äžã€ã§ãã \(\phi^e\) ãçšããŠGNNã¬ã€ã€ãŒãå®çŸ©ããŸãã
ããã§æ±ãååã°ã©ãã¯ç¡åã°ã©ããªã®ã§ãã©ã®ããŒãã \(\vec{v}_{rk}\) ãåä¿¡ããã©ã®ããŒãã \(\vec{v}_{sk}\) ãéä¿¡ããããã©ã®ããã«æ±ºããã°è¯ãã§ããããïŒ ããããã® \(\vec{e}^{'}_k\) ã¯æ¬¡ã®ã¹ãããã§ããŒã \(v_{rk}\) ãžã®å ¥åãšããŠéçŽãããŸããååã°ã©ãã§ã¯ãå šãŠã®çµåã¯ååããã®ãå ¥åããšãåºåãã®äž¡æ¹ãå Œãããããå šãŠã®çµåã2ã€ã®æåãšããžãšããŠåãæ±ãããšã«ããŸãïŒä»ã«è¯ãæ¹æ³ããªãã®ã§ãïŒïŒC-Hçµåã¯CããHãžã®èŸºãšHããCãžã®ãšããžã§æ§æãããããšã«ãªããŸããæåã®çåã«æ»ããŸããã \(\vec{v}_{rk} \)ãš \(\vec{v}_{sk}\) ãšã¯äœã§ããããïŒé£æ¥è¡åã®ãã¹ãŠã®èŠçŽ ïŒ\(k\)ïŒãèãã\(k = \{ij\}\) ããªãã¡èŠçŽ \(A_{ij}\) ã«ã€ããŠã¯ãåä¿¡ããŒãã \(j\) ãéä¿¡ããŒãã \(i\) ã§ããããšã衚ããŸããéåãã®ãšããžã«ããã飿¥è¡åã®èŠçŽ \(A_{ji}\) ãèãããšãåä¿¡ããŒãã \(i\) ãéä¿¡ããŒãã \(j\) ãšãªããŸãã
\(\vec{e}^{'}_k\) ã¯GCNããã®ã¡ãã»ãŒãžã®ãããªãã®ã§ãããããäžè¬çã§ãåä¿¡ããŒããšã°ã©ãç¹åŸŽãã¯ãã« \(\vec{u}\) ã®æ å ±ãåæ ããããšãã§ããŸããæ¥åžžçãªæå³ã§ã®ãã¡ãã»ãŒãžãã¯äžåºŠéä¿¡ãããã°èª°ïŒãããã¯äœïŒã«åä¿¡ããããã«ãã£ãŠå 容ãå€ããããã§ã¯ãªãã®ã§ã \(\vec{e}^{'}_k\) ãã¡ãã»ãŒãžã®æ¯å©ã§èª¬æããããšãããšããããªããšã«ãªããŸãããšããããæ°ãããšããžã®æŽæ°ã¯ãæåã®éçŽé¢æ°ã§éçŽãããŸãïŒ
\(\rho^{e\rightarrow v}\) ã¯æã ãå®çŸ©ãã颿°ã \(E_i^{'}\) ã¯ããŒã \(i\) ã« åãã å šãŠã®ãšããžããã® \(\vec{e}^{'}_k\) ãã¹ã¿ãã¯ãããã®ã§ããéçŽããããšããžã䜿ã£ãŠãããŒãã®æŽæ°ãèšç®ã§ããŸãïŒ
以äžã§æ°ããããŒããšãšããžãåŸãããã®ã§ãGNNã¬ã€ã€ãŒã®éåžžã®ã¹ãããã¯å®äºã§ããããã°ã©ãç¹åŸŽ (\(\vec{u}\)) ãæŽæ°ããå Žåã以äžã®ã¹ãããã远å ã§å®çŸ©ãããããšããããŸãïŒ
ãã®åŒã¯ãã°ã©ãå šäœã«ã€ããŠå šãŠã®ã¡ãã»ãŒãž/éçŽããããšããžãéçŽããŸããããã«ãããæ°ããããŒããã°ã©ãå šäœã«ã€ããŠéçŽã§ããŸãïŒ
ãããŠæåŸã«ã次ã®ããã«ããŠã°ã©ãç¹åŸŽãã¯ãã«ãæŽæ°ã§ããŸãïŒ
8.10.1. Battaglia equationsã«ããGCNã®åå®åŒå¶
Battagliaã®åŒã«ããGCNãã©ã®ããã«èšè¿°ããããèŠãŠã¿ãŸãããããŸã (8.8) ã䜿ã£ãŠã飿¥ããå¯èœæ§ã®ãããã¹ãŠã®é£æ¥ããŒãã«å¯ŸããŠã¡ãã»ãŒãžãèšç®ããŸããGCNã§ã¯ãã¡ãã»ãŒãžã¯éä¿¡è ã«ã®ã¿äŸåããåä¿¡è ã«ã¯äŸããªãããšã«æ³šæããŠãã ããã
(8.9) ã«ãããŠããŒã \(i\) ã«ãã£ãŠããã¡ãã»ãŒãžãéçŽããããã«ããããã®ã¡ãã»ãŒãžã®å¹³åããšããŸãïŒ
ç¶ããŠãããŒãã®æŽæ°ãè¡ããŸãããããã¯åã«ã¡ãã»ãŒãžã«ã€ããŠæŽ»æ§å颿°ãé©çšããã ãã§ã (8.10)
äžåŒã«ãã㊠\(\sigma(\bar{e}^{'}_i + \vec{v}_i)\) ãšå€æŽãå ããããšã§ãã°ã©ãã«èªå·±ã«ãŒããæãããããšãå¯èœã§ããGCNã§ã¯ä»ã®é¢æ°ã¯å¿ èŠãªãã®ã§ãããã3ã€ã®åŒã ãã§GCNãå®çŸ©ããããšãã§ããŸãã
8.11. The SchNet Architecture¶
æãå€ãããã€ããçšããããGNNã®1ã€ã«ãSchNetãããã¯ãŒã¯ [SchuttSK+18] ããããŸããçºè¡šåœæã¯ããŸãGNNãšããŠã¯èªèãããŠããŸããã§ããããçŸåšã§ã¯ãã®äžã€ãšããŠèªèãããããŒã¹ã©ã€ã³ã¢ãã«ãšããŠãã䜿ãããŠããŸããããŒã¹ã©ã€ã³ã¢ãã«ãšã¯ãæ°ææ³ãšã®æ¯èŒã«çšããããã¢ãã«ã®ããšã§ãåºãåãå ¥ãããããã€æ§ã ãªå®éšãéããŠè¯ãæ§èœã瀺ãããšã確èªãããŠããã¢ãã«ã䜿ãããŸãã
ãããŸã§ã«æ±ã£ãå šãŠã®äŸã§ã¯ãååãã°ã©ããšããŠã¢ãã«ãžå ¥åããŠããŸãããäžæ¹SchNetã§ã¯ãååã°ã©ãã§ã¯ãªããååãxyz座æšïŒç¹ïŒãšããŠè¡šçŸãå ¥åããxyz座æšãã°ã©ãã«å€æããããšã§GNNNãé©çšããŸããSchNetã¯ãçµåæ å ±ãªãã§ååã®é 眮ã®ã¿ãããšãã«ã®ãŒãåãäºæž¬ããããã«éçºãããŸããããããã£ãŠãSchNetãçè§£ããããã«ããŸãååãšãã®äœçœ®ã®ã»ãããã©ã®ããã«ã°ã©ãã«å€æããããã確èªããŸããããåååãããŒãåããæé ã¯ç°¡åã§ãäžèšãšåæ§ã®åŠçãè¡ã£ãåŸãååçªå·ãembeddingã¬ã€ã€ãŒã«æž¡ããŸããããã¯ãåååçªå·ã«åŠç¿å¯èœãªãã¯ãã«ãå²ãåœãŠãããšïŒè𳿳šïŒåŠç¿ã«ãããåååã®æœè±¡çç¹åŸŽãæãã髿¬¡å ãã¯ãã«ãåŸãããšïŒãæå³ããŸãïŒembeddingã«ã€ããŠã®åŸ©ç¿ã¯ Standard Layers ãåç §ããŠãã ããïŒã
飿¥è¡åã®èšç®ãç°¡åã§ãå šãŠã®ååãå šãŠã®ååã«æ¥ç¶ãããããã«ããã ãã§ããåã«å šååãçžäºã«æ¥ç¶ããã®ã ãšããããGNNã䜿ãæå³ãããããããªããšæããããããããŸããããã®ãããªæäœãããçç±ã¯ãGNNã¯é åºç䟡ã§ããããã§ããããååãxyz座æšãšããŠåŠç¿ããããšãããšïŒååã®äžŠã³æ¹ã«ãã£ãŠéã¿ãå€ãã£ãŠããŸãäžã«ãæ§é ããšã®ååæ°ã®éããããŸãåãæ±ããªãããšã§ãããã
SchNetãçè§£ããããã«ããäžã€æŒãããã¹ãç¹ã¯ãåååã®xyz座æšã®æ å ±ã¯ã©ãæ±ãããã®ãããšããããšã§ããSchNetã§ã¯ãxyz座æšãããšããžç¹åŸŽãæ§ç¯ããããšã«ãããã¢ãã«ã«åº§æšã®æ å ±ãåã蟌ãã§ããŸããåå \(i\) ãš \(j\) ã®éã®ãšããž \(\vec{e}\) ã¯ãã·ã³ãã«ã«ãããã®ååéè·é¢ \(r\) ããèšç®ãããŸãã
\(\gamma\) ã¯ãã€ããŒãã©ã¡ãŒã¿ïŒäŸïŒ 10Ã
ïŒ \(\mu_k\) 㯠[0, 5, 10, 15 , 20]
ã®ãããªã¹ã«ã©ãŒã®çééã°ãªããã§ãã (8.14) ã®æäœã¯ãååçªå·ãå
±æçµåã®çš®é¡ã®ãããªã«ããŽãªç¹åŸŽãone-hotãã¯ãã«ã«å€æããããšã«äŒŒãŠããŸããããããã«ããŽãªã«ã«ãªéãšç°ãªããè·é¢ã¯é£ç¶å€ã§ç¡éã«ããã®ã§ãone-hotãã¯ãã«ãšããŠè¡šçŸããããšã¯ã§ããŸãããããã§ãäžçš®ã®ãã¹ã ãŒãžã³ã°ãã«ãã£ãŠãè·é¢ãæ¬äŒŒçã«one-hot衚çŸããŠããã®ã§ãããã®æèŠãã€ããããã«ãäŸãèŠãŠã¿ãŸãããïŒ
gamma = 1
mu = np.linspace(0, 10, 5)
def rbf(r):
return np.exp(-gamma * (r - mu) ** 2)
print("input", 2)
print("output", np.round(rbf(2), 2))
input 2
output [0.02 0.78 0. 0. 0. ]
è·é¢ã\(r=2\) ã¯ã \(k = 1\)ãã®äœçœ®ïŒè𳿳šïŒkã¯ãã®listã®indexã®ããšã§ãïŒãåŒ·ãæŽ»æ§åãããã¯ãã«ãäžããããšãããããŸãããã㯠\(\mu_1 = 2\) ã§ããããšã«å¯Ÿå¿ããŸãã
ãããŸã§ã§ããŒããšãšããžããã³ãGNNã®æŽæ°æ¹çšåŒãå®çŸ©ããŸãããããã«ããå°ãã ãèšå·ãå®çŸ©ããŠããå¿ èŠããããŸããããã§ã¯ãMLPïŒMultilayer perceptronïŒã衚ãããã« \(h(\vec{x})\) ã䜿çšããŸããMLPã¯ãåºæ¬çã«1å±€ãããã¯2å±€ã®å šçµåã¬ã€ã€ãŒãããªããã¥ãŒã©ã«ãããã¯ãŒã¯ã§ãããããã®MLPã«ãããå šçµåã¬ã€ã€ãŒã®æ£ç¢ºãªæ°ãããã€ã»ã©ãã§æŽ»æ§åãè¡ãããšãã£ã詳现ã¯ãéèŠãªç¹ãçè§£ããäžã§äžèŠãªãã説æã¯çç¥ããŸãïŒãããã®è©³çްã¯ä»¥äžã®å®è£ äŸãåç §ããŠãã ããïŒãã§ã¯ããã§ãå šçµåã¬ã€ã€ãŒã®å®çŸ©ãæãåºããŸãããïŒ
ãŸããSchNetã§ã¯ âshifted softplusâ ãšåŒã°ããæ°ããªæŽ»æ§å颿° \(\sigma\) ãçšããŸãïŒ \(\sigma = \ln\left(0.5e^{x} + 0.5\right)\) ã Fig. 8.4 ã«ãããŠã \(\sigma(x)\) ãšéåžžã®ReLU掻æ§åãæ¯èŒããåæãå ±åãããŠããŸããshifted softplusã䜿ãçç±ã¯ãå ¥åã«å¯ŸããŠæ»ããã§ããããã§ãããã®ãããç²åå士ã®è·é¢ïŒpairwise distanceïŒã«å¯ŸããŠæ»ãããªåŸ®åãå¿ èŠãšãããåååååŠã·ãã¥ã¬ãŒã·ã§ã³ã®ãããªã¢ããªã±ãŒã·ã§ã³ã«ãããŠããã©ãŒã¹ïŒè𳿳šïŒç²åå士ã«åãåïŒãèšç®ããããã«SchNetã䜿ãããšãã§ããŸãã
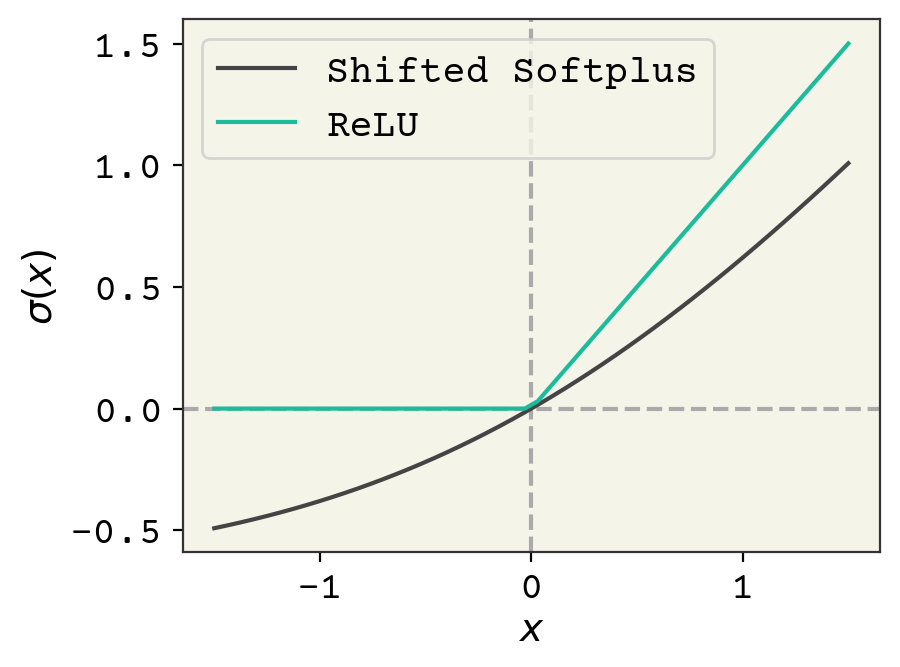
Fig. 8.4 äžè¬çãªReLU掻æ§å颿°ãšãSchNetã§äœ¿çšãããŠããshifted softplusã®æ¯èŒÂ¶
ããŠãå眮ããç¶ããŸããããããããGNNæ¹çšåŒã«è©±ãç§»ããŸãããšããžã®æŽæ°æ¹çšåŒ (8.8) ã¯2ã€ã®éšåããæããŸãããŸãããã£ãŠãããšããžïŒïŒçµåïŒç¹åŸŽãšãããŒãïŒïŒååïŒã®ç¹åŸŽãMLPã«éããŸããç¶ããŠããããã®çµæã次ã®MLPã«éããŸãïŒ
次ã«ãšããžã®éçŽé¢æ° (8.9) ãèããŸããããSchNetã§ã¯ããšããžéçŽã¯è¿åã®ååç¹åŸŽéã«å¯Ÿããåã§ãã
æåŸã«ãSchNetã®ããŒãæŽæ°é¢æ°ã¯ä»¥äžã®ããã«ãªããŸãïŒ
éåžžãGNNã®æŽæ°ã¯3ã6åé©çšãããŸããäžèšã®SchNetã®èª¬æã«ãããŠããšããžã®æŽæ°åŒãå®çŸ©ããŸããããGCNåæ§ã«å®éã«ã¯ãšããžç¹åŸŽãäžæžããããåå±€ã§åããšããžç¹åŸŽãä¿ãããŸããå ã ã®SchNetã¯ãšãã«ã®ãŒãåãäºæž¬ããããã®ãã®ãªã®ã§ãèªã¿åºãã¯sum-poolingãäžèšã®ãããªæŠç¥ã§è¡ãããšãå¯èœã§ãã
ãããã®åŒã®è©³çްã¯å€æŽãããããšããããŸããããªãªãžãã«ã®SchNetã®è«æã§ã¯ã \(h_1\) ã¯æŽ»æ§åãªãã®denseãª1å±€ã\(h_2\) ã¯æŽ»æ§åããã®2å±€ã\(h_3\) ã¯1å±€ã«æŽ»æ§åããã»2å±€ç®ã¯æŽ»æ§åç¡ãã®denseãª2å±€ã®æ§æãçšããããŸããã
SchNetãšã¯?
SchNetããŒã¹ã®GNNã®äž»ãªç¹åŸŽã¯ãïŒ1ïŒãšããžã®æŽæ°ïŒã¡ãã»ãŒãžã®çµã¿ç«ãŠïŒã«ãšããžãšããŒãã®ç¹åŸŽãçšããããšã§ãïŒ
ããã§ \(h_i()\) ã¯äœããã®åŠç¿å¯èœãªé¢æ°ã§ããç¹åŸŽïŒ2ïŒã¯ãããŒãæŽæ°ã«æ®å·®ãå©çšããããšã§ãïŒ
ãã®ä»ããšããžç¹åŸŽã®äœãæ¹ã\(h_i\) ã®å±€æ°ã掻æ§å颿°ã®éžæãèªã¿åºãã®æ¹æ³ããã€ã³ãã¯ã©ãŠããã°ã©ãã«å€æããæ¹æ³ãªã©ã詳现ã¯å šãŠ [SchuttSK+18] ã§ææ¡ãããSchNetã¢ãã«ã®å®çŸ©ã«æºæ ããŸãã
8.12. SchNet Example: Predicting Space Groups¶
Our next example will be a SchNet model that predict space groups of points. Identifying the space group of atoms is an important part of crystal structure identification, and when doing simulations of crystallization. Our SchNet model will take as input points and output the predicted space group. This is a classification problem; specifically it is multi-class becase a set of points should only be in one space group. To simplify our plots and analysis, we will work in 2D where there are 17 possible space groups.
Our data for this is a set of points from various point groups. The features are xyz coordinates and the label is the space group. We will not have multiple atom types for this problem. The hidden cell below loads the data and reshapes it for the example.
import gzip
import pickle
import urllib
urllib.request.urlretrieve(
"https://github.com/whitead/dmol-book/raw/master/data/sym_trajs.pb.gz",
"sym_trajs.pb.gz",
)
with gzip.open("sym_trajs.pb.gz", "rb") as f:
trajs = pickle.load(f)
label_str = list(set([k.split("-")[0] for k in trajs]))
# now build dataset
def generator():
for k, v in trajs.items():
ls = k.split("-")[0]
label = label_str.index(ls)
traj = v
for i in range(traj.shape[0]):
yield traj[i], label
data = tf.data.Dataset.from_generator(
generator,
output_signature=(
tf.TensorSpec(shape=(None, 2), dtype=tf.float32),
tf.TensorSpec(shape=(), dtype=tf.int32),
),
).shuffle(1000)
# The shuffling above is really important because this dataset is in order of labels!
val_data = data.take(100)
test_data = data.skip(100).take(100)
train_data = data.skip(200)
Letâs take a look at a few examples from the dataset
The Data
This data was generated from [CW22] and all points are constrained to match the space group exactly during a molecular dynamics simulation. The trajectories were NPT with a positive pressure and followed the procedure in that paper for Figure 2. The force field is Lennard-Jones with \(\sigma=1\) and \(\epsilon=1\)
fig, axs = plt.subplots(4, 5, figsize=(12, 8))
axs = axs.flatten()
# get a few example and plot them
for i, (x, y) in enumerate(data):
if i == 20:
break
axs[i].plot(x[:, 0], x[:, 1], ".")
axs[i].set_title(label_str[y.numpy()])
axs[i].axis("off")
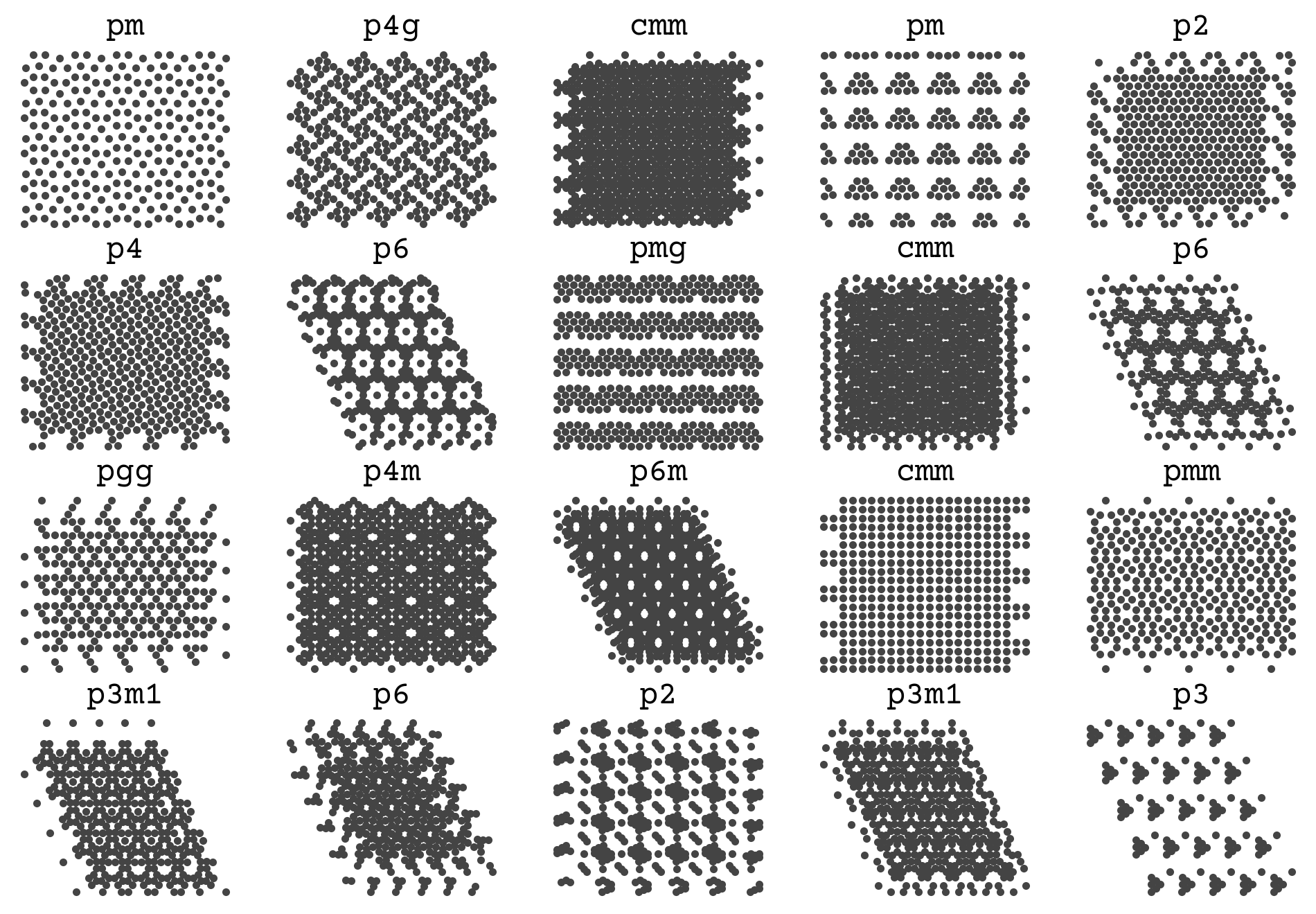
You can see that there is a variable number of points and a few examples for each space group. The goal is to infer those titles on the plot from the points alone.
8.12.1. Building the graphs¶
We now need to build the graphs for the points. The nodes are all identical - so they can just be 1s (weâll reserve 0 in case we want to mask or pad at some point in the future). As described in the SchNet section above, the edges should be distance to every other atom. In most implementations of SchNet, we practically add a cut-off on either distance or maximum degree (edges per node). Weâll do maximum degree for this work of 16.
I have a function below that is a bit sophisticated. It takes a matrix of point positions in arbitrary dimension and returns the distances and indices to the nearest k
neighbors - exactly what we need. It uses some tricks from Tensors and Shapes. However, it is not so important for you to understand this function. Just know it takes in points and gives us the edge features and edge nodes.
# this decorator speeds up the function by "compiling" it (tracing it)
# to run efficienty
@tf.function(
reduce_retracing=True,
)
def get_edges(positions, NN, sorted=True):
M = tf.shape(input=positions)[0]
# adjust NN
NN = tf.minimum(NN, M)
qexpand = tf.expand_dims(positions, 1) # one column
qTexpand = tf.expand_dims(positions, 0) # one row
# repeat it to make matrix of all positions
qtile = tf.tile(qexpand, [1, M, 1])
qTtile = tf.tile(qTexpand, [M, 1, 1])
# subtract them to get distance matrix
dist_mat = qTtile - qtile
# mask distance matrix to remove zros (self-interactions)
dist = tf.norm(tensor=dist_mat, axis=2)
mask = dist >= 5e-4
mask_cast = tf.cast(mask, dtype=dist.dtype)
# make masked things be really far
dist_mat_r = dist * mask_cast + (1 - mask_cast) * 1000
topk = tf.math.top_k(-dist_mat_r, k=NN, sorted=sorted)
return -topk.values, topk.indices
Letâs see how this function works by showing the connections between points in one of our examples. Iâve hidden the code below. It shows some pointâs neighbors and connects them so you can get a sense of how a set of points is converted into a graph. The complete graph will have all pointsâ neighborhoods.
from matplotlib import collections
fig, axs = plt.subplots(2, 3, figsize=(12, 8))
axs = axs.flatten()
for i, (x, y) in enumerate(data):
if i == 6:
break
e_f, e_i = get_edges(x, 8)
# make things easier for plotting
e_i = e_i.numpy()
x = x.numpy()
y = y.numpy()
# make lines from origin to its neigbhors
lines = []
colors = []
for j in range(0, x.shape[0], 23):
# lines are [(xstart, ystart), (xend, yend)]
lines.extend([[(x[j, 0], x[j, 1]), (x[k, 0], x[k, 1])] for k in e_i[j]])
colors.extend([f"C{j}"] * len(e_i[j]))
lc = collections.LineCollection(lines, linewidths=2, colors=colors)
axs[i].add_collection(lc)
axs[i].plot(x[:, 0], x[:, 1], ".")
axs[i].axis("off")
axs[i].set_title(label_str[y])
plt.show()
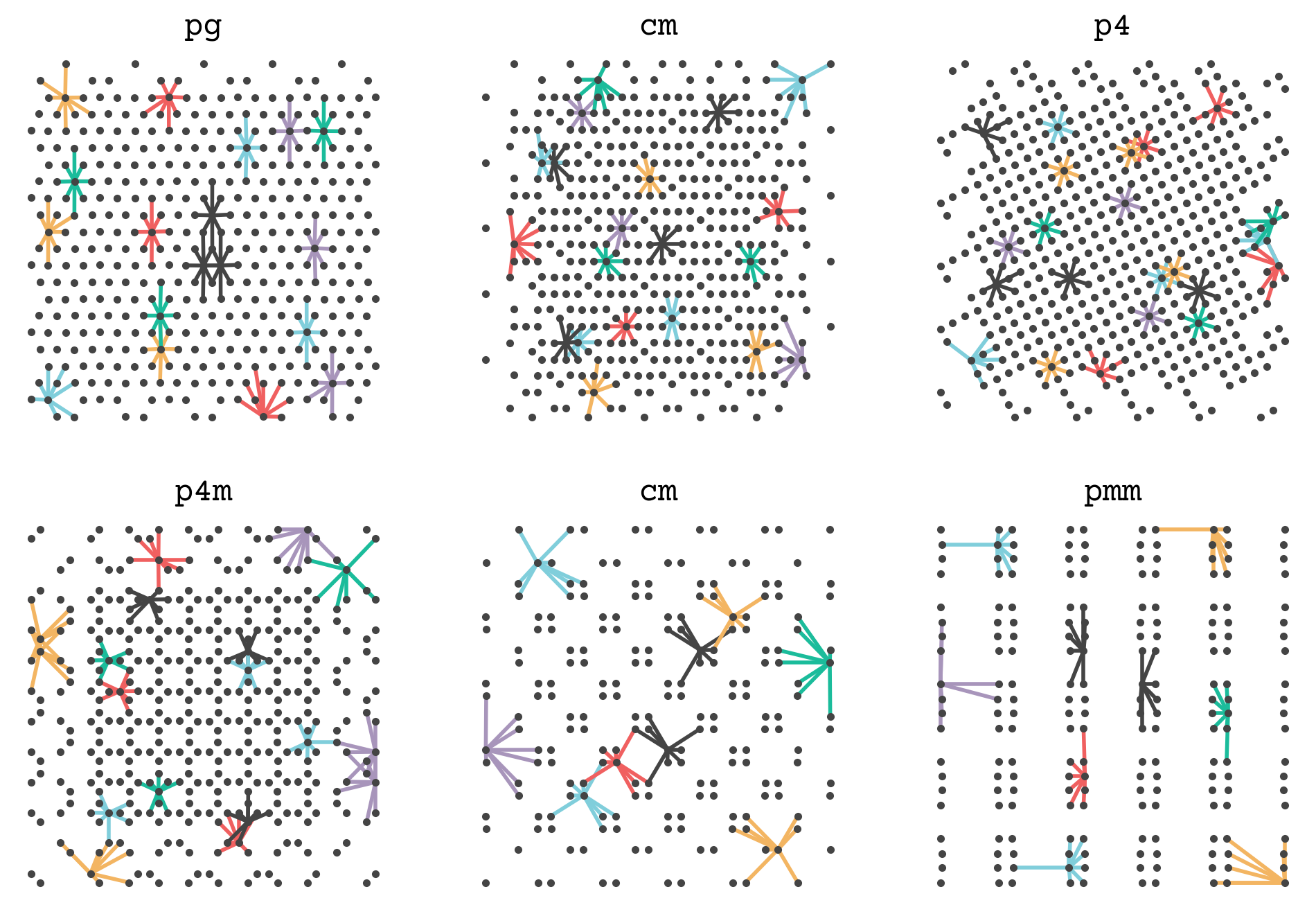
We will now add this function and the edge featurization of SchNet (8.14) to get the graphs for the GNN steps.
MAX_DEGREE = 16
EDGE_FEATURES = 8
MAX_R = 20
gamma = 1
mu = np.linspace(0, MAX_R, EDGE_FEATURES)
def rbf(r):
return tf.exp(-gamma * (r[..., tf.newaxis] - mu) ** 2)
def make_graph(x, y):
edge_r, edge_i = get_edges(x, MAX_DEGREE)
edge_features = rbf(edge_r)
return (tf.ones(tf.shape(x)[0], dtype=tf.int32), edge_features, edge_i), y[None]
graph_train_data = train_data.map(make_graph)
graph_val_data = val_data.map(make_graph)
graph_test_data = test_data.map(make_graph)
Letâs examine one graph to see what it looks like. Weâll slice out only the first nodes.
for (n, e, nn), y in graph_train_data:
print("first node:", n[1].numpy())
print("first node, first edge features:", e[1, 1].numpy())
print("first node, all neighbors", nn[1].numpy())
print("label", y.numpy())
break
first node: 1
first node, first edge features: [2.8479335e-01 4.9036104e-02 6.8545725e-10 7.7790052e-25 0.0000000e+00
0.0000000e+00 0.0000000e+00 0.0000000e+00]
first node, all neighbors [ 7 11 10 206 4 12 197 9 13 15 3 192 200 2 130 195]
label [13]
8.12.2. Implementing the MLPs¶
Now we can implement the SchNet model! Letâs start with the \(h_1,h_2,h_3\) MLPs that are used in the GNN update equations. In the SchNet paper these each had different numbers of layers and different decisions about which layers had activation. Letâs create them now.
def ssp(x):
# shifted softplus activation
return tf.math.log(0.5 * tf.math.exp(x) + 0.5)
def make_h1(units):
return tf.keras.Sequential([tf.keras.layers.Dense(units)])
def make_h2(units):
return tf.keras.Sequential(
[
tf.keras.layers.Dense(units, activation=ssp),
tf.keras.layers.Dense(units, activation=ssp),
]
)
def make_h3(units):
return tf.keras.Sequential(
[tf.keras.layers.Dense(units, activation=ssp), tf.keras.layers.Dense(units)]
)
One detail that can be missed is that the weights in each MLP should change in each layer of SchNet. Thus, weâve written the functions above to always return a new MLP. This means that a new set of trainable weights is generated on each call, meaning there is no way we could erroneously have the same weights in multiple layers.
8.12.3. Implementing the GNN¶
Now we have all the pieces to make the GNN. This code will be very similar to the GCN example above, except we now have edge features. One more detail is that our readout will be an MLP as well, following the SchNet paper. The only change weâll make is that we want our output property to be (1) multi-class classification and (2) intensive (independent of number of atoms). So weâll end with an average (intensive) and end with an output vector of logits the size of our labels.
class SchNetModel(tf.keras.Model):
"""Implementation of SchNet Model"""
def __init__(self, gnn_blocks, channels, label_dim, **kwargs):
super(SchNetModel, self).__init__(**kwargs)
self.gnn_blocks = gnn_blocks
# build our layers
self.embedding = tf.keras.layers.Embedding(2, channels)
self.h1s = [make_h1(channels) for _ in range(self.gnn_blocks)]
self.h2s = [make_h2(channels) for _ in range(self.gnn_blocks)]
self.h3s = [make_h3(channels) for _ in range(self.gnn_blocks)]
self.readout_l1 = tf.keras.layers.Dense(channels // 2, activation=ssp)
self.readout_l2 = tf.keras.layers.Dense(label_dim)
def call(self, inputs):
nodes, edge_features, edge_i = inputs
# turn node types as index to features
nodes = self.embedding(nodes)
for i in range(self.gnn_blocks):
# get the node features per edge
v_sk = tf.gather(nodes, edge_i)
e_k = self.h1s[i](v_sk) * self.h2s[i](edge_features)
e_i = tf.reduce_sum(e_k, axis=1)
nodes += self.h3s[i](e_i)
# readout now
nodes = self.readout_l1(nodes)
nodes = self.readout_l2(nodes)
return tf.reduce_mean(nodes, axis=0)
Remember that the key attributes of a SchNet GNN are the way that we use edge and node features. We can see the mixing of these two in the key line for computing the edge update (computing message values):
e_k = self.h1s[i](v_sk) * self.h2s[i](edge_features)
followed by aggregation of the edges updates (pooling messages):
e_i = tf.reduce_sum(e_k, axis=1)
and the node update
nodes += self.h3s[i](e_i)
Also of note is how we go from node features to multi-classs. We use dense layers that get the shape per-node into the number of classes
self.readout_l1 = tf.keras.layers.Dense(channels // 2, activation=ssp)
self.readout_l2 = tf.keras.layers.Dense(label_dim)
and then we take the average over all nodes
return tf.reduce_mean(nodes, axis=0)
Letâs give now use the model on some data.
small_schnet = SchNetModel(3, 32, len(label_str))
for x, y in graph_train_data:
yhat = small_schnet(x)
break
print(yhat.numpy())
[ 0.01385795 0.00906286 0.00222304 -0.00563365 0.00490831 0.00621619
0.02778318 0.0169939 -0.00951115 -0.00167371 -0.0171227 -0.00270679
-0.00358437 0.00626842 -0.00611755 0.01474886 0.01494901]
The output is the correct shape and remember it is logits. To get a class prediction that sums to probability 1, we need to use a softmax:
print("predicted class", tf.nn.softmax(yhat).numpy())
predicted class [0.05939336 0.05910925 0.05870633 0.0582469 0.05886419 0.05894123
0.06022622 0.05957991 0.05802149 0.05847801 0.05758153 0.05841763
0.05836639 0.0589443 0.05821873 0.0594463 0.0594582 ]
8.12.4. Training¶
Great! It is untrained though. Now we can set-up training. Our loss will be cross-entropy from logits, but we need to be careful on the form. Our labels are integers - which is called âsparseâ labels because they are not full one-hots. Mult-class classification is also known as categorical classification. Thus, the loss we want is sparse categorical cross entropy from logits.
small_schnet.compile(
optimizer=tf.keras.optimizers.Adam(1e-4),
loss=tf.keras.losses.SparseCategoricalCrossentropy(from_logits=True),
metrics="sparse_categorical_accuracy",
)
result = small_schnet.fit(graph_train_data, validation_data=graph_val_data, epochs=20)
plt.plot(result.history["sparse_categorical_accuracy"], label="training accuracy")
plt.plot(result.history["val_sparse_categorical_accuracy"], label="validation accuracy")
plt.axhline(y=1 / 17, linestyle="--", label="random")
plt.legend()
plt.xlabel("Epoch")
plt.ylabel("Accuracy")
plt.show()
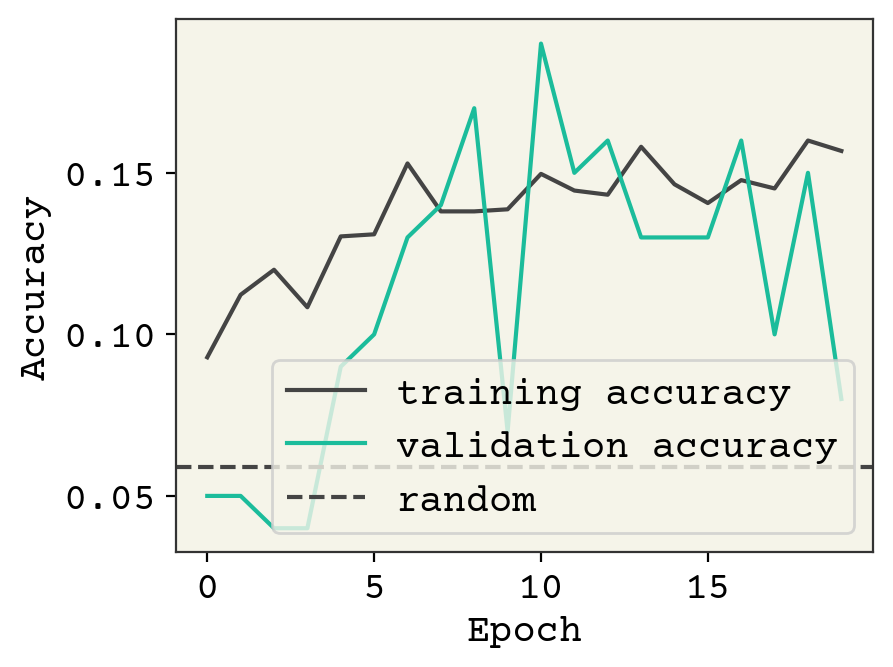
The accuracy is not great, but it looks like we could keep training. We have a very small SchNet here. Standard SchNet described in [SchuttSK+18] uses 6 layers and 64 channels and 300 edge features. We have 3 layers and 32 channels. Nevertheless, weâre able to get some learning. Letâs visually see whatâs going on with the trained model on some test data
fig, axs = plt.subplots(4, 5, figsize=(12, 8))
axs = axs.flatten()
for i, ((x, y), (gx, _)) in enumerate(zip(test_data, graph_test_data)):
if i == 20:
break
axs[i].plot(x[:, 0], x[:, 1], ".")
yhat = small_schnet(gx)
yhat_i = tf.math.argmax(tf.nn.softmax(yhat)).numpy()
axs[i].set_title(f"True: {label_str[y.numpy()]}\nPredicted: {label_str[yhat_i]}")
axs[i].axis("off")
plt.tight_layout()
plt.show()
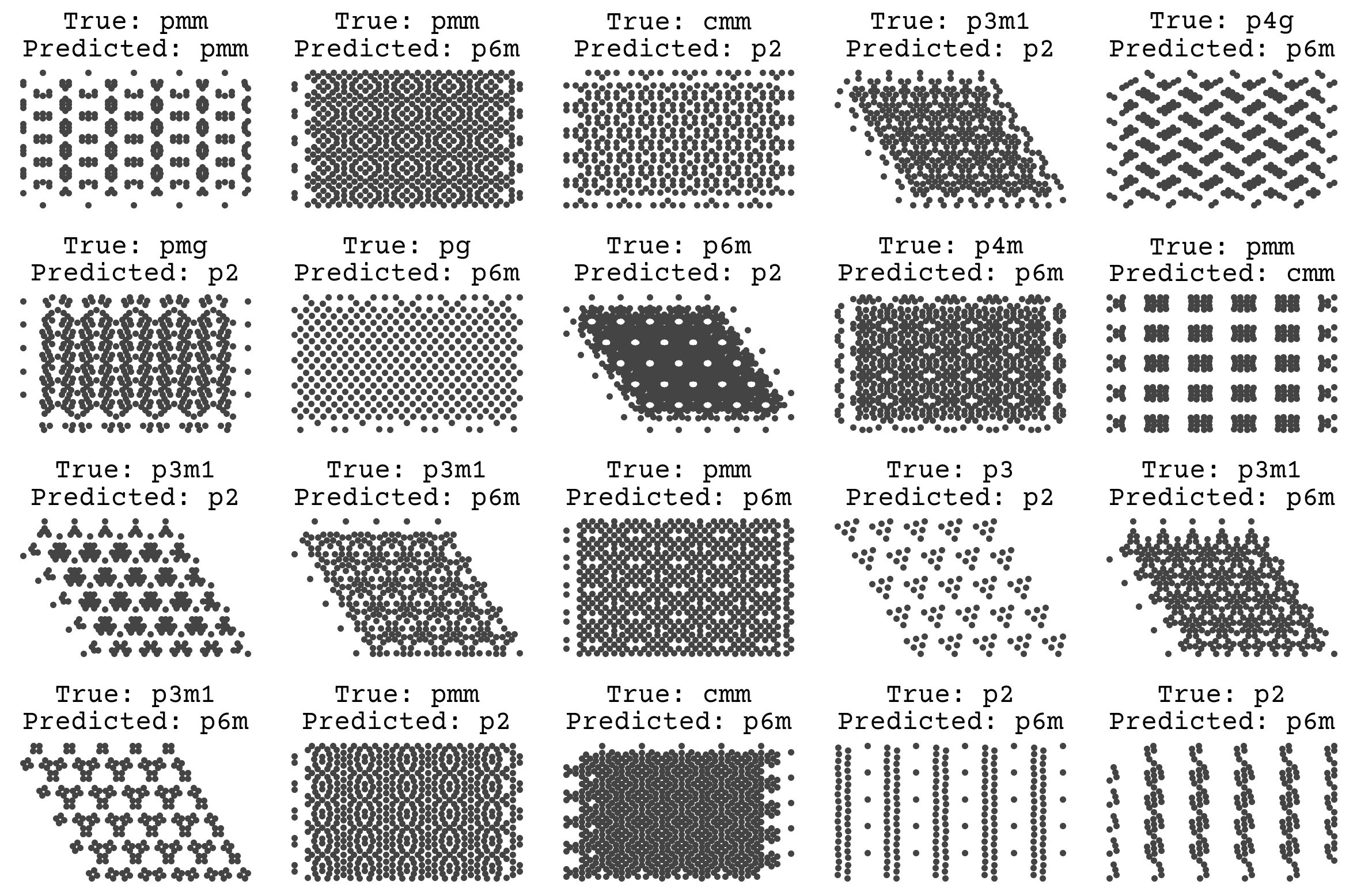
Weâll revisit this example later! One unique fact about this dataset is that it is synthetic, meaning there is no label noise. As discussed in Regression & Model Assessment, that removes the possibility of overfitting and leads us to favor high variance models. The goal of teaching a model to predict space groups is to apply it on real simulations or microscopy data, which will certainly have noise. We could have mimicked this by adding noise to the labels in the data and/or by randomly removing atoms to simulate defects. This would better help our model work in a real setting.
8.13. Current Research Directions¶
8.13.1. Common Architecture Motifs and Comparisons¶
Weâve now seen message passing layer GNNs, GCNs, GGNs, and the generalized Battaglia equations. Youâll find common motifs in the architectures, like gating, ã¢ãã³ã·ã§ã³ã¬ã€ã€ãŒ, and pooling strategies. For example, Gated GNNS (GGNs) can be combined with attention pooling to create Gated Attention GNNs (GAANs)[ZSX+18]. GraphSAGE is a similar to a GCN but it samples when pooling, making the neighbor-updates of fixed dimension[HYL17]. So youâll see the suffix âsageâ when you sample over neighbors while pooling. These can all be represented in the Battaglia equations, but you should be aware of these names.
The enormous variety of architectures has led to work on identifying the âbestâ or most general GNN architecture [DJL+20, EPBM19, SMBGunnemann18]. Unfortunately, the question of which GNN architecture is best is as difficult as âwhat benchmark problems are best?â Thus there are no agreed-upon conclusions on the best architecture. However, those papers are great resources on training, hyperparameters, and reasonable starting guesses and I highly recommend reading them before designing your own GNN. There has been some theoretical work to show that simple architectures, like GCNs, cannot distinguish between certain simple graphs [XHLJ18]. How much this practically matters depends on your data. Ultimately, there is so much variety in hyperparameters, data equivariances, and training decisions that you should think carefully about how much the GNN architecture matters before exploring it with too much depth.
8.13.2. Nodes, Edges, and Features¶
Youâll find that most GNNs use the node-update equation in the Battaglia equations but do not update edges. For example, the GCN will update nodes at each layer but the edges are constant. Some recent work has shown that updating edges can be important for learning when the edges have geometric information, like if the input graph is a molecule and the edges are distance between the atoms [KGrossGunnemann20]. As weâll see in the chapter on equivariances (Input Data & Equivariances), one of the key properties of neural networks with point clouds (i.e., Cartesian xyz coordinates) is to have rotation equivariance. [KGrossGunnemann20] showed that you can achieve this if you do edge updates and encode the edge vectors using a rotation equivariant basis set with spherical harmonics and Bessel functions. These kind of edge updating GNNs can be used to predict protein structure [JES+20].
Another common variation on node features is to pack more into node features than just element identity. In many examples, you will see people inserting valence, elemental mass, electronegativity, a bit indicating if the atom is in a ring, a bit indicating if the atom is aromatic, etc. Typically these are unnecessary, since a model should be able to learn any of these features which are computed from the graph and node elements. However, we and others have empirically found that some can help, specifically indicating if an atom is in a ring [LWC+20]. Choosing extra features to include though should be at the bottom of your list of things to explore when designing and using GNNs.
8.13.3. Beyond Message Passing¶
One of the common themes of GNN research is moving âbeyond message passing,â where message passing is the message construction, aggregation, and node update with messages. Some view this as impossible â claiming that all GNNs can be recast as message passing [Velivckovic22]. Another direction is on disconnecting the underlying graph being input to the GNN and the graph used to compute updates. We sort of saw this above with SchNet, where we restricted the maximum degree for the message passing. More useful are ideas like âliftingâ the graphs into more structured objects like simplicial complexes [BFO+21]. Finally, you can also choose where to send the messages beyond just neighbors [TZK21]. For example, all nodes on a path could communicate messages or all nodes in a clique.
8.13.4. Do we need graphs?¶
It is possible to convert a graph into a string if youâre working with an adjacency matrix without continuous values. Molecules specifically can be converted into a string. This means you can use layers for sequences/strings (e.g., recurrent neural networks or 1D convolutions) and avoid the complexities of a graph neural network. SMILES is one way to convert molecular graphs into strings. With SMILES, you cannot predict a per-atom quantity and thus a graph neural network is required for atom/bond labels. However, the choice is less clear for per-molecule properties like toxicity or solubility. There is no consensus about if a graph or string/SMILES representation is better. SMILES can exceed certain graph neural networks in accuracy on some tasks. SMILES is typically better on generative tasks. Graphs obviously beat SMILES in label representations, because they have granularity of bonds/edges. Weâll see how to model SMILES in Deep Learning on Sequences, but it is an open question of which is better.
8.13.5. Stereochemistry/Chiral Molecules¶
Stereochemistry is fundamentally a 3D property of molecules and thus not present in the covalent bonding. It is measured experimentally by seeing if molecules rotate polarized light and a molecule is called chiral or âoptically activeâ if it is experimentally known to have this property. Stereochemistry is the categorization of how molecules can preferentially rotate polarized light through asymmetries with respect to their mirror images. In organic chemistry, the majority of stereochemistry is of enantiomers. Enantiomers are âhandednessâ around specific atoms called chiral centers which have 4 or more different bonded atoms. These may be treated in a graph by indicating which nodes are chiral centers (nodes) and what their state or mixture of states (racemic) are. This can be treated as an extra processing step. Amino acids and thus all proteins are entaniomers with only one form present. This chirality of proteins means many drug molecules can be more or less potent depending on their stereochemistry.
Fig. 8.5 This is a molecule with axial stereochemistry. Its small helix could be either left or right-handed.¶
Adding node labels is not enough generally. Molecules can interconvert between stereoisomers at chiral centers through a process called tautomerization. There are also types of stereochemistry that are not at a specific atom, like rotamers that are around a bond. Then there is stereochemistry that involves multiple atoms like axial helecene. As shown in Fig. 8.5, the molecule has no chiral centers but is âoptically activeâ (experimentally measured to be chiral) because of its helix which can be left- or right-handed.
8.14. Relevant Videos¶
8.14.1. Intro to GNNs¶
8.14.2. Overview of GNN with Molecule, Compiler Examples¶
8.15. Chapter Summary¶
Molecules can be represented by graphs by using one-hot encoded feature vectors that show the elemental identity of each node (atom) and an adjacency matrix that show immediate neighbors (bonded atoms).
Graph neural networks are a category of deep neural networks that have graphs as inputs.
One of the early GNNs is the Kipf & Welling GCN. The input to the GCN is the node feature vector and the adjacency matrix, and returns the updated node feature vector. The GCN is permutation invariant because it averages over the neighbors.
A GCN can be viewed as a message-passing layer, in which we have senders and receivers. Messages are computed from neighboring nodes, which when aggregated update that node.
A gated graph neural network is a variant of the message passing layer, for which the nodes are updated according to a gated recurrent unit function.
The aggregation of messages is sometimes called pooling, for which there are multiple reduction operations.
GNNs output a graph. To get a per-atom or per-molecule property, use a readout function. The readout depends on if your property is intensive vs extensive
The Battaglia equations encompasses almost all GNNs into a set of 6 update and aggregation equations.
You can convert xyz coordinates into a graph and use a GNN like SchNet
8.16. Cited References¶
- DJL+20(1,2)
Vijay Prakash Dwivedi, Chaitanya K Joshi, Thomas Laurent, Yoshua Bengio, and Xavier Bresson. Benchmarking graph neural networks. arXiv preprint arXiv:2003.00982, 2020.
- BBL+17
Michael M Bronstein, Joan Bruna, Yann LeCun, Arthur Szlam, and Pierre Vandergheynst. Geometric deep learning: going beyond euclidean data. IEEE Signal Processing Magazine, 34(4):18â42, 2017.
- WPC+20
Zonghan Wu, Shirui Pan, Fengwen Chen, Guodong Long, Chengqi Zhang, and SÂ Yu Philip. A comprehensive survey on graph neural networks. IEEE Transactions on Neural Networks and Learning Systems, 2020.
- LWC+20(1,2)
Zhiheng Li, Geemi P Wellawatte, Maghesree Chakraborty, Heta A Gandhi, Chenliang Xu, and Andrew D White. Graph neural network based coarse-grained mapping prediction. Chemical Science, 11(35):9524â9531, 2020.
- YCW20
Ziyue Yang, Maghesree Chakraborty, and Andrew D White. Predicting chemical shifts with graph neural networks. bioRxiv, 2020.
- XFLW+19
Tian Xie, Arthur France-Lanord, Yanming Wang, Yang Shao-Horn, and Jeffrey C Grossman. Graph dynamical networks for unsupervised learning of atomic scale dynamics in materials. Nature communications, 10(1):1â9, 2019.
- SLRPW21
Benjamin Sanchez-Lengeling, Emily Reif, Adam Pearce, and Alex Wiltschko. A gentle introduction to graph neural networks. Distill, 2021. https://distill.pub/2021/gnn-intro. doi:10.23915/distill.00033.
- XG18
Tian Xie and Jeffrey C. Grossman. Crystal graph convolutional neural networks for an accurate and interpretable prediction of material properties. Phys. Rev. Lett., 120:145301, Apr 2018. URL: https://link.aps.org/doi/10.1103/PhysRevLett.120.145301, doi:10.1103/PhysRevLett.120.145301.
- KW16
Thomas N Kipf and Max Welling. Semi-supervised classification with graph convolutional networks. arXiv preprint arXiv:1609.02907, 2016.
- GSR+17
Justin Gilmer, Samuel S Schoenholz, Patrick F Riley, Oriol Vinyals, and George E Dahl. Neural message passing for quantum chemistry. arXiv preprint arXiv:1704.01212, 2017.
- LTBZ15
Yujia Li, Daniel Tarlow, Marc Brockschmidt, and Richard Zemel. Gated graph sequence neural networks. arXiv preprint arXiv:1511.05493, 2015.
- CGCB14
Junyoung Chung, Caglar Gulcehre, KyungHyun Cho, and Yoshua Bengio. Empirical evaluation of gated recurrent neural networks on sequence modeling. arXiv preprint arXiv:1412.3555, 2014.
- XHLJ18(1,2)
Keyulu Xu, Weihua Hu, Jure Leskovec, and Stefanie Jegelka. How powerful are graph neural networks? In International Conference on Learning Representations. 2018.
- LDLio19
Enxhell Luzhnica, Ben Day, and Pietro Liò. On graph classification networks, datasets and baselines. arXiv preprint arXiv:1905.04682, 2019.
- MSK20
Diego Mesquita, Amauri Souza, and Samuel Kaski. Rethinking pooling in graph neural networks. Advances in Neural Information Processing Systems, 2020.
- GZBA21
Daniele Grattarola, Daniele Zambon, Filippo Maria Bianchi, and Cesare Alippi. Understanding pooling in graph neural networks. arXiv preprint arXiv:2110.05292, 2021.
- DRA21
Ameya Daigavane, Balaraman Ravindran, and Gaurav Aggarwal. Understanding convolutions on graphs. Distill, 2021. https://distill.pub/2021/understanding-gnns. doi:10.23915/distill.00032.
- ZKR+17(1,2)
Manzil Zaheer, Satwik Kottur, Siamak Ravanbakhsh, Barnabas Poczos, Russ R Salakhutdinov, and Alexander J Smola. Deep sets. In Advances in neural information processing systems, 3391â3401. 2017.
- BHB+18
Peter W Battaglia, Jessica B Hamrick, Victor Bapst, Alvaro Sanchez-Gonzalez, Vinicius Zambaldi, Mateusz Malinowski, Andrea Tacchetti, David Raposo, Adam Santoro, Ryan Faulkner, and others. Relational inductive biases, deep learning, and graph networks. arXiv preprint arXiv:1806.01261, 2018.
- SchuttSK+18(1,2,3)
Kristof T SchÃŒtt, Huziel E Sauceda, P-J Kindermans, Alexandre Tkatchenko, and K-R MÃŒller. Schnetâa deep learning architecture for molecules and materials. The Journal of Chemical Physics, 148(24):241722, 2018.
- CW22
Sam Cox and Andrew D White. Symmetric molecular dynamics. arXiv preprint arXiv:2204.01114, 2022.
- ZSX+18
Jiani Zhang, Xingjian Shi, Junyuan Xie, Hao Ma, Irwin King, and Dit-Yan Yeung. Gaan: gated attention networks for learning on large and spatiotemporal graphs. arXiv preprint arXiv:1803.07294, 2018.
- HYL17
Will Hamilton, Zhitao Ying, and Jure Leskovec. Inductive representation learning on large graphs. In Advances in neural information processing systems, 1024â1034. 2017.
- EPBM19
Federico Errica, Marco Podda, Davide Bacciu, and Alessio Micheli. A fair comparison of graph neural networks for graph classification. In International Conference on Learning Representations. 2019.
- SMBGunnemann18
Oleksandr Shchur, Maximilian Mumme, Aleksandar Bojchevski, and Stephan GÃŒnnemann. Pitfalls of graph neural network evaluation. arXiv preprint arXiv:1811.05868, 2018.
- KGrossGunnemann20(1,2)
Johannes Klicpera, Janek GroÃ, and Stephan GÃŒnnemann. Directional message passing for molecular graphs. In International Conference on Learning Representations. 2020.
- JES+20
Bowen Jing, Stephan Eismann, Patricia Suriana, Raphael JL Townshend, and Ron Dror. Learning from protein structure with geometric vector perceptrons. arXiv preprint arXiv:2009.01411, 2020.
- Velivckovic22
Petar VeliÄkoviÄ. Message passing all the way up. arXiv preprint arXiv:2202.11097, 2022.
- BFO+21
Cristian Bodnar, Fabrizio Frasca, Nina Otter, Yuguang Wang, Pietro Lio, Guido F Montufar, and Michael Bronstein. Weisfeiler and lehman go cellular: cw networks. Advances in Neural Information Processing Systems, 34:2625â2640, 2021.
- TZK21
Erik Thiede, Wenda Zhou, and Risi Kondor. Autobahn: automorphism-based graph neural nets. Advances in Neural Information Processing Systems, 2021.